Born to be cloud
Creating robust digital systems that flourish in an evolving landscape. Our services, spanning from Cloud to Applications, Data, and AI, are trusted by 150+ customers. Collaborating with our global partners, we transform possibilities into tangible outcomes.
.png)
.png)
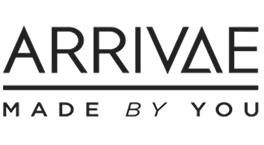
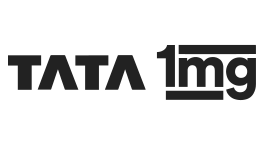
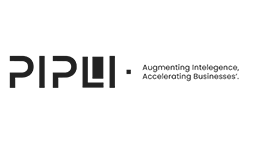
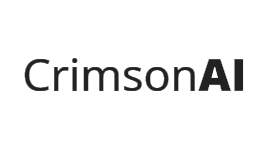
.png)
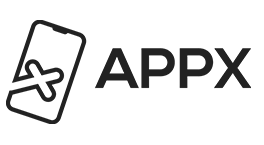

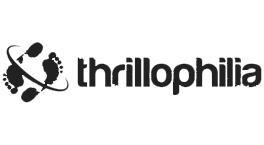
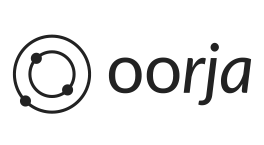
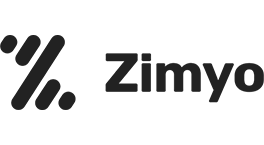
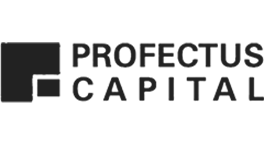
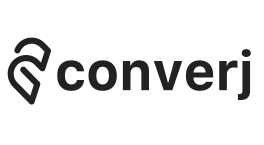
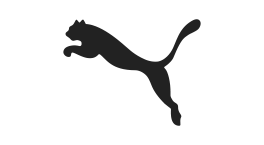

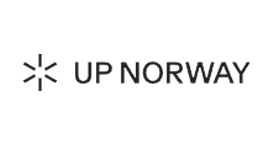
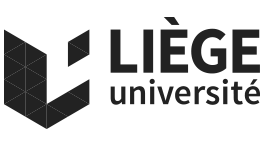
.png)
.png)
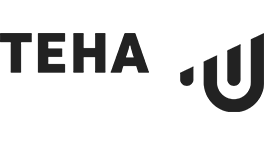

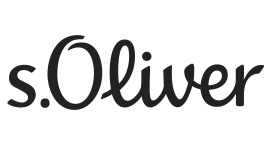
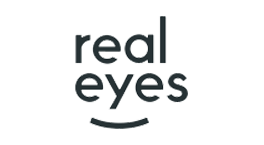
.png)
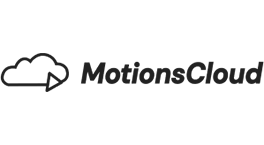
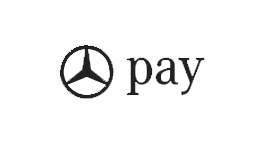
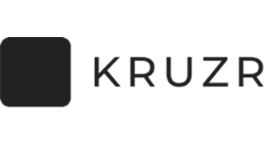

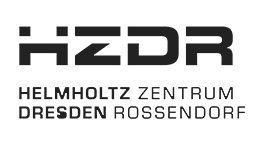
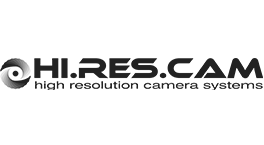
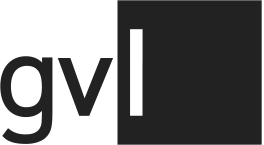
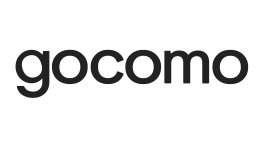

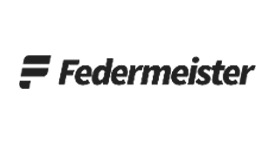
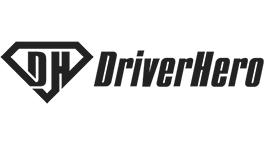
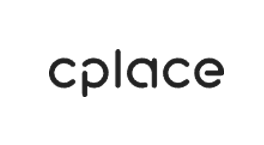
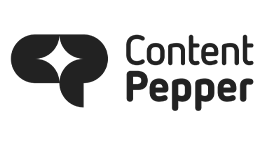
.png)
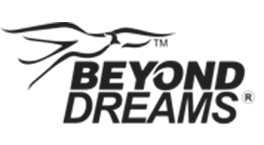
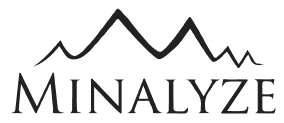
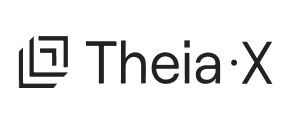
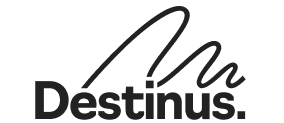
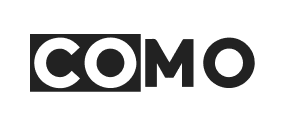
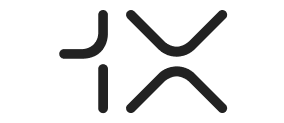
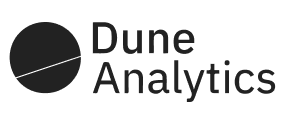
.png)
.png)
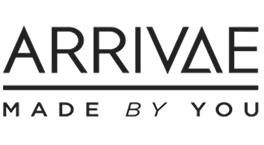
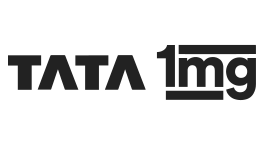
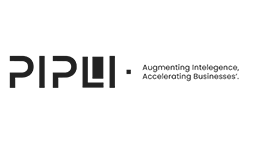
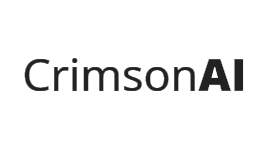
.png)
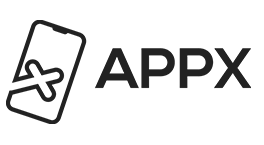

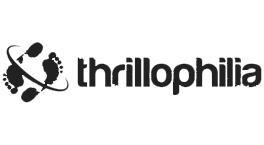
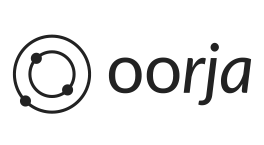
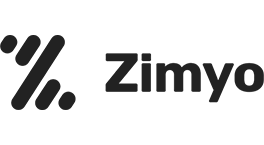
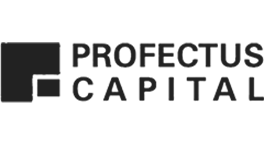
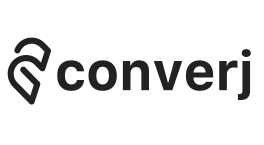
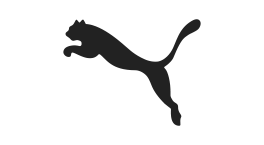

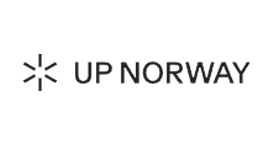
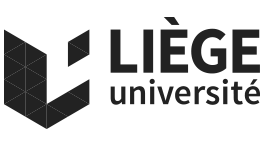
.png)
.png)
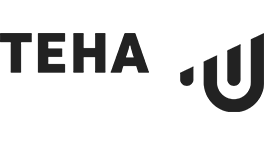

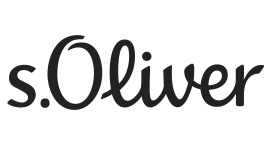
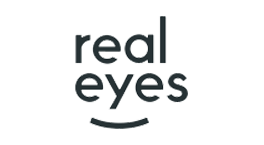
.png)
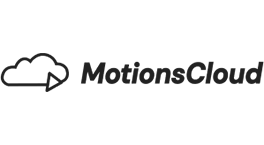
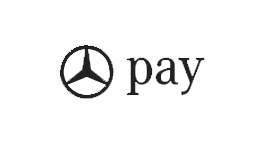
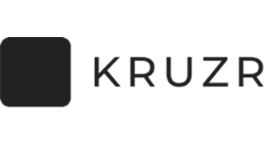

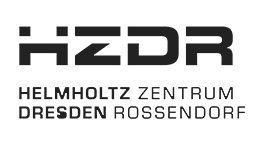
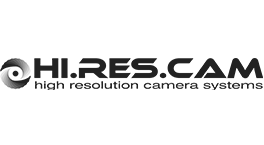
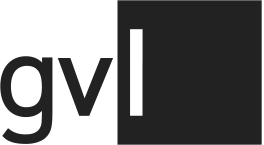
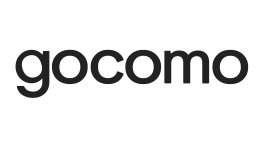

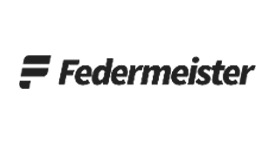
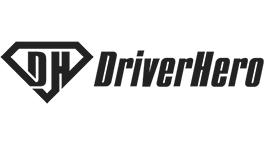
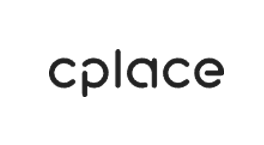
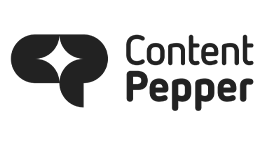
.png)
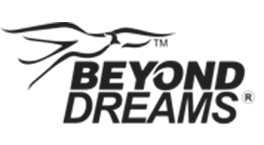
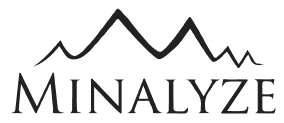
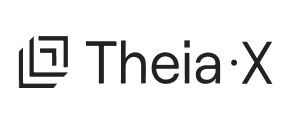
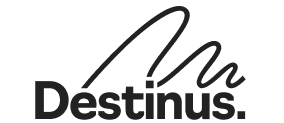
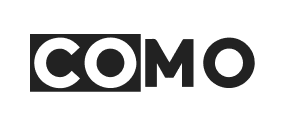
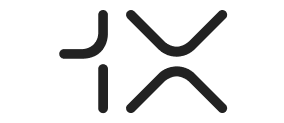
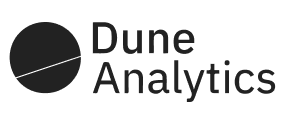
.png)
.png)
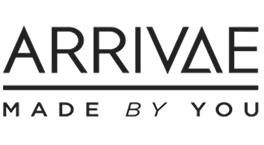
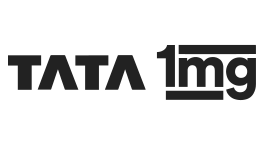
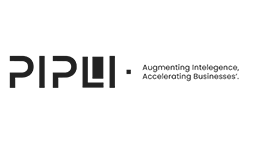
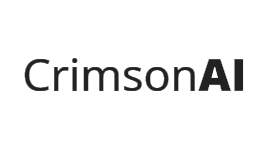
.png)
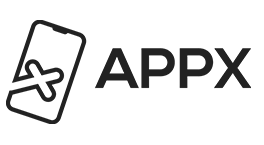

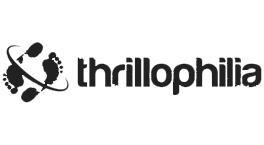
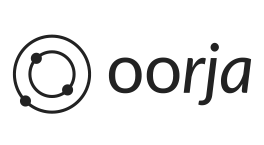
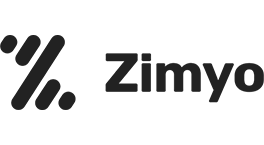
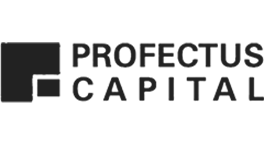
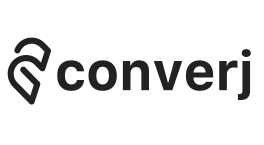
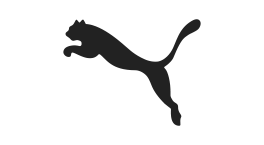

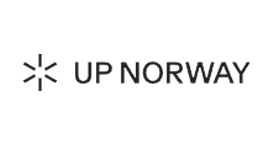
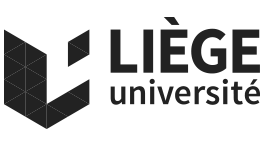
.png)
.png)
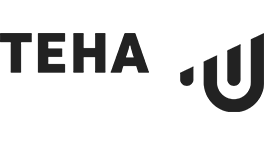

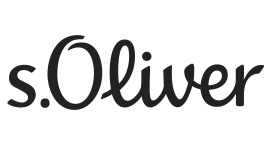
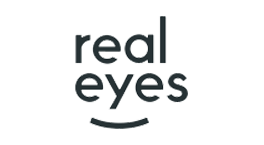
.png)
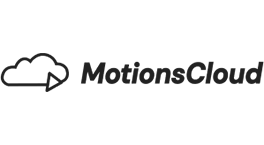
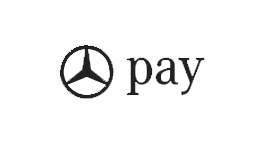
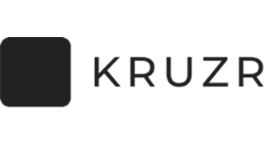

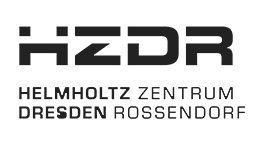
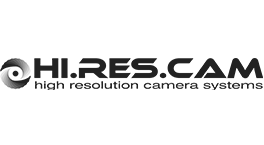
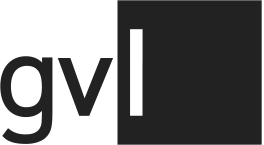
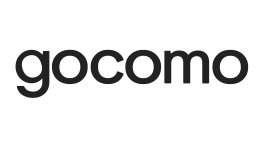

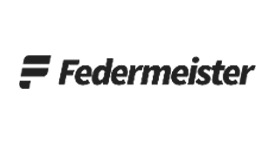
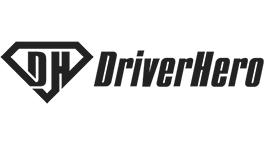
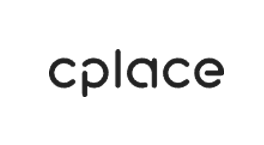
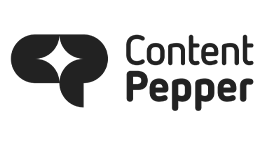
.png)
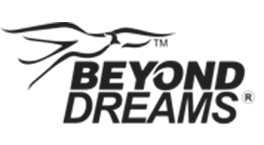
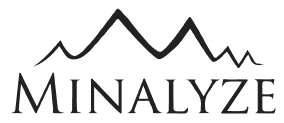
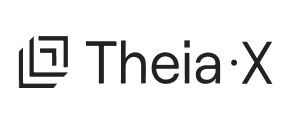
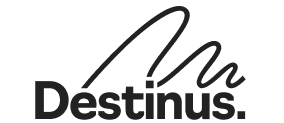
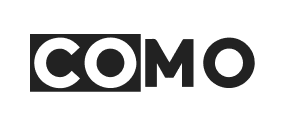
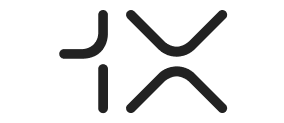
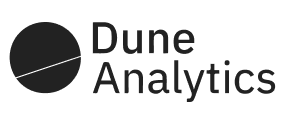
.png)
.png)
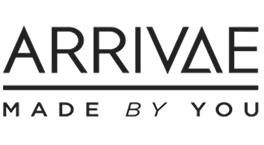
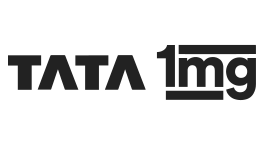
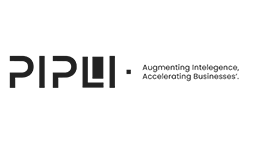
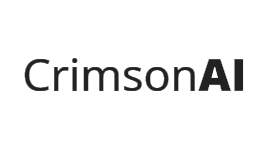
.png)
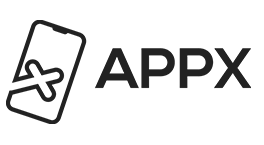

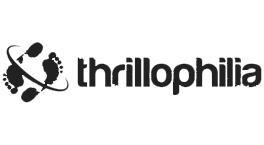
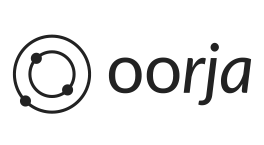
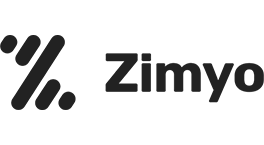
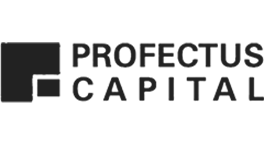
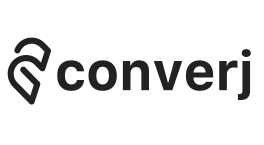
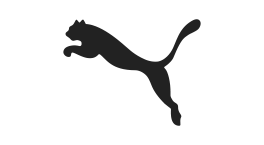

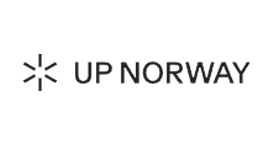
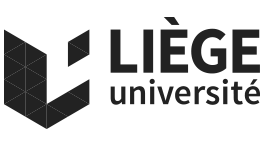
.png)
.png)
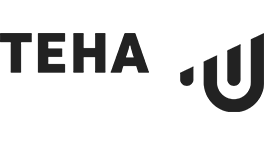

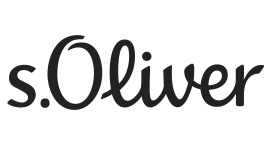
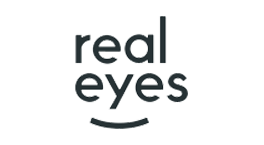
.png)
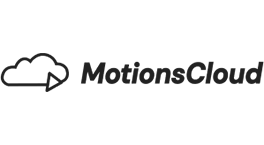
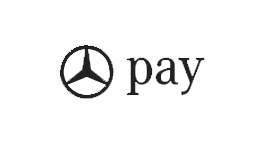
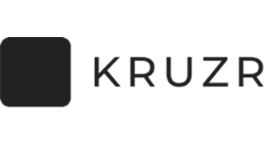

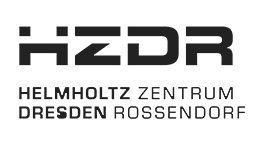
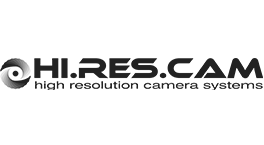
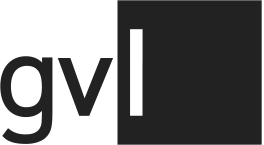
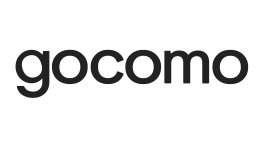

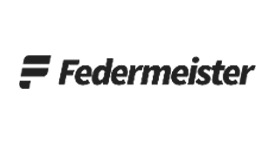
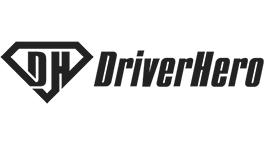
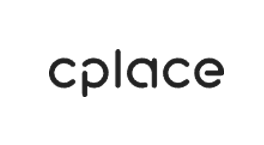
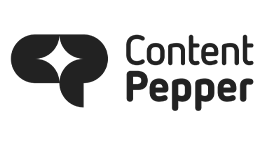
.png)
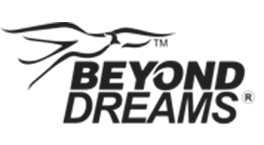
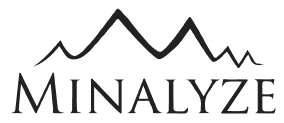
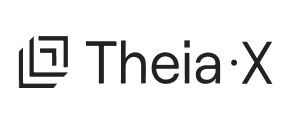
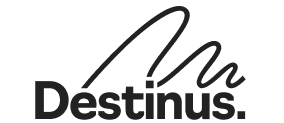
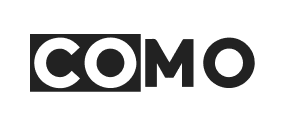
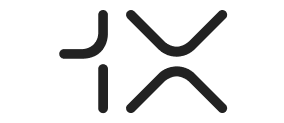
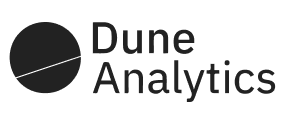
Experience our services.
We can help to make the move - design, built and migrate to the cloud.
Cloud Migration
Maximise your investment in the cloud and achieve cost-effectiveness, on-demand scalability, unlimited computing, and enhanced security.
Artificial Intelligence/ Machine Learning
Infuse AI & ML into your business to solve complex problems, drive top-line growth, and innovate mission critical applications.
Data & Analytics
Discover the Hidden Gems in Your Data with cloud-native Analytics. Our comprehensive solutions cover data processing, analysis, and visualization.
Generative Artificial Intelligence (GenAI)
Drive measurable business success with GenAI, Where creative solutions lead to tangible outcomes, including improved operational efficiency, enhanced customer satisfactions, and accelerated time-to-market.
.png)
.png)
Competencies

-p-500.png)
-p-500.png)
.png)
Awards
.png)
.png)
Ankercloud: Partners with AWS, GCP, and Azure
We excel through partnerships with industry giants like AWS, GCP, and Azure, offering innovative solutions backed by leading cloud technologies.


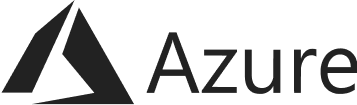
Countless Happy Clients and Counting!
.png)
.png)
Check out our blog

Email Spoofing: What is it and How to Protect Against it
E-mail spoofing is a technique whereby the attacker forges the sender's address in such a way as to make the recipient believe it is coming from a trusted party. It could be a colleague in the office, a company, or even a financial institution. In this scenario, an attacker sends email headers in a manner such that the recipient would consider it to have come from a particular person, and the possibility of getting people to click on links they should not download attachments that will harm them or share with sensitive information becomes more probable.
Most of the current email systems lack a good feature that identifies forged sender addresses, and this explains why most phishing, spear-phishing, and business email compromise attacks rely on such a strategy.
For example, you might get an email that you are in both "To" and "From." Now, that doesn't mean someone has breached your email account. What they would do instead is pretend to be you or maybe another person you trust so that you open the email and may click on malicious links or release sensitive information.
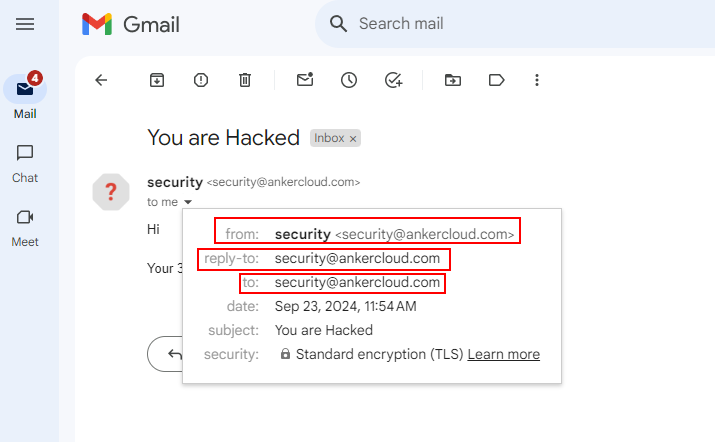
How to Identify a Spoofed Email?
Sent Folder does not have an e-mail - When you send yourself an e-mail, it always is going to be in your Sent folder. And consequently, since spammers spoofed your address, you'd never see it there because he sent it from nowhere, but it would appear as if it was from your account.


NOTE: If you compose an email to yourself and delete it from your sent folder, then you will no longer see the email in your inbox also.
Check the Original Email - Most E-mail systems will show you the "original" or "raw" version of an e-mail. This shows all the technical details of how the e-mail is sent. Look for a possible sign such as suspicious From, Reply-To, Return-Path, or Received, fields that don't look just right, maybe an indicator of a spoofed e-mail.
Grammar and spelling mistakes - Always keep an eye out for homoglyphs (Example: Ankercloud as Amk3rc10ud) and grammatical errors that a legitimate sender would never make.
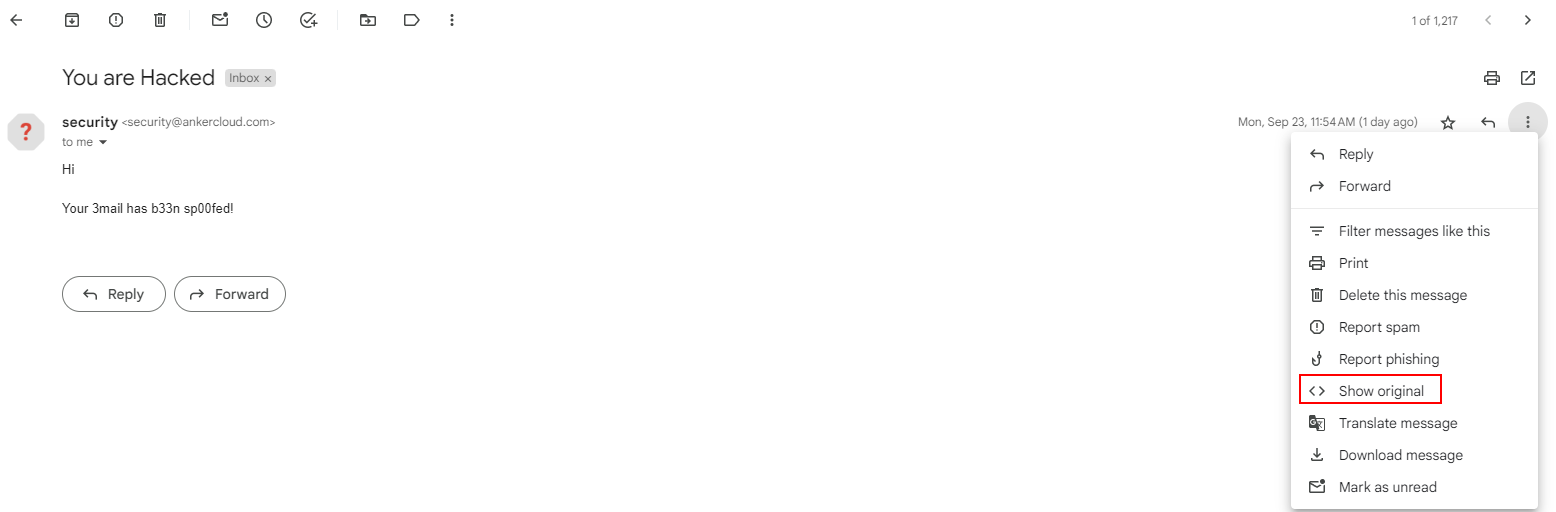
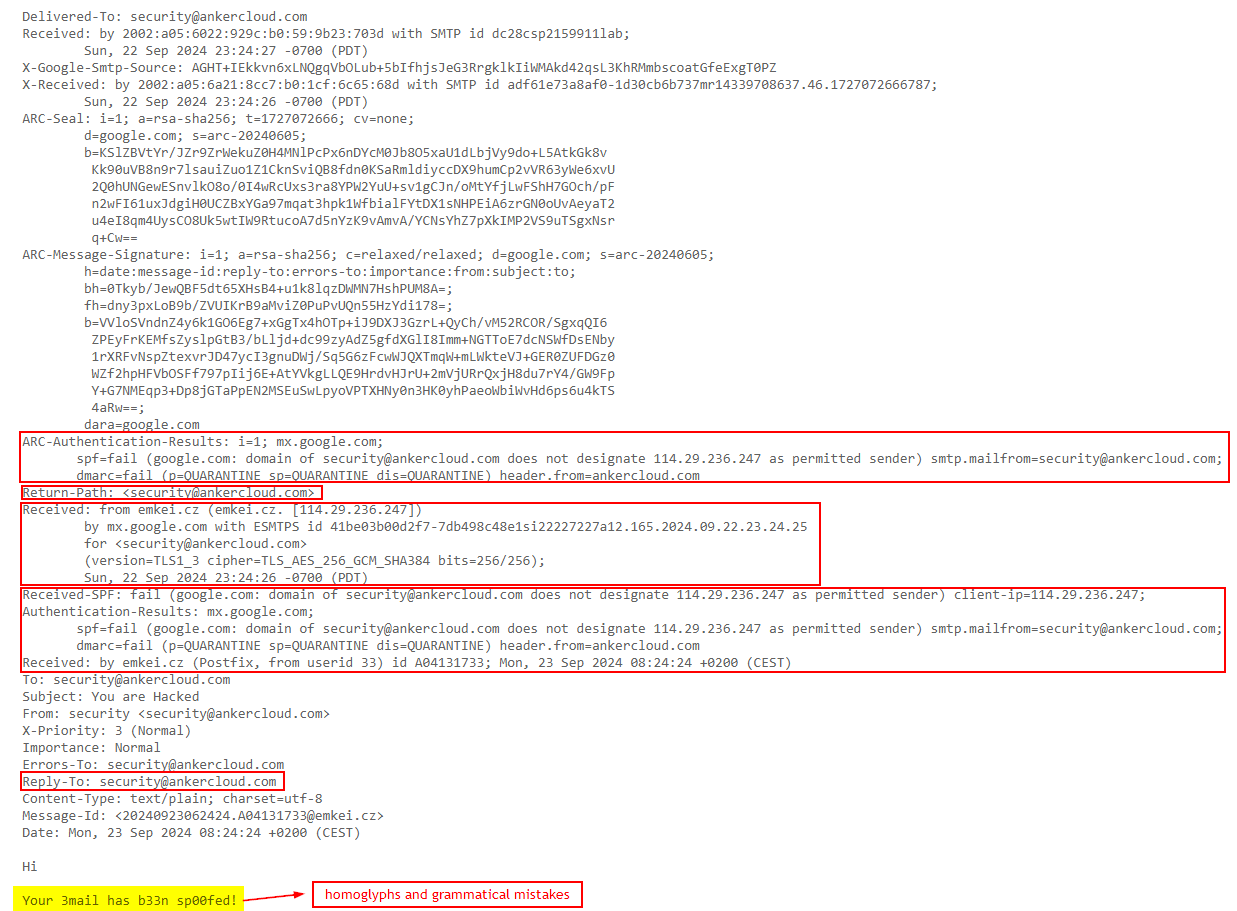
Preventive measures: protecting both yourself and your organization
- Implement the SPF, DKIM, and DMARC email security protocols as a layer of protection against spoofing which helps in verifying that an email was actually sent from the person that it says it is, if not then the email will go to spam/deleted immediately.
- Use spam filters- Generally, most email services have spam filters. These can filter suspicious-looking emails even before they reach your inbox. To be on the safe side, assume that your spam filter is active.
- Be Cautious - Be wary of opening any uncalled-for emails, particularly when suspicious, or asking for information that could be confidential.
What to Do If You Fall Victim to Spoofing Attack
- Don’t panic and disconnect the device from the internet immediately, to make sure no further damage is done by the malicious programs downloaded.
- Report the IT team on what happened so they can decide if sensitive information has been put at risk and make all the necessary arrangements to ensure safety measures are taken about your account.
- Change your passwords in case you think you got infected, change all the passwords right away from a different device, and implement/enforce 2FA for that added layer of security.
- Keep an eye on your email account and other accounts online for suspicious activities such as unauthorized login attempts or suspicious activities through your accounts.
Conclusion
Email spoofing is very hard to prevent, but definitely difficult to detect; if you are alert and think of some basic precautions, you should never fall for such a scam. So, always check suspicious emails and contact your IT/security team whenever in doubt, please ask for help.
2

Navigating the New AI Act in Europe: How Ankercloud can help your business to comply with it
In June 2023, the European Union took a significant step in regulating artificial intelligence with the introduction of the AI Act. This landmark legislation aims to create a legal framework for AI development and deployment across the EU, ensuring that AI technologies are used responsibly and ethically. For businesses leveraging AI, understanding and complying with the AI Act is crucial to avoid penalties and maintain consumer trust. This is where Ankercloud comes into play, offering solutions to help businesses navigate the complexities of the new law.
Understanding the AI Act
The AI Act classifies AI systems into three categories based on their potential risks:
- Unacceptable Risk: AI applications deemed a threat to safety, livelihoods, and rights are banned. This includes systems for social scoring by governments and real-time biometric identification in public spaces.
- High Risk: AI systems that impact critical areas such as healthcare, transport, and employment must meet strict requirements. This includes robust risk management, high-quality data, human oversight, and transparency.
- Limited Risk: Applications with a minimal risk, like chatbots, must ensure transparency and inform users that they are interacting with AI.
For businesses, especially those operating in high-risk categories, the AI Act mandates a comprehensive approach to risk management, data governance, and compliance documentation.
Challenges for Businesses
The introduction of the AI Act presents several challenges for businesses:
- Compliance Costs: Adhering to the stringent requirements of the AI Act may involve significant costs, particularly for SMEs.
- Technical Expertise: Ensuring that AI systems meet the law's standards requires specialized knowledge in AI development and data governance.
- Documentation and Transparency: Businesses need to maintain detailed records of their AI systems' design, testing, and deployment processes.
How Ankercloud Can Help
Ankercloud offers a suite of services designed to help businesses comply with the new AI Act seamlessly and efficiently. Here’s how Ankercloud can assist:
- Risk Assessment and Management:
- Comprehensive Risk Analysis: Ankercloud provides support to assess the risks associated with AI systems, helping businesses identify potential issues early in the development process in line with the industry best practices.
- Risk Mitigation Strategies: Through detailed analysis and expert guidance, Ankercloud helps implement robust risk mitigation strategies, ensuring compliance with the AI Act’s high-risk requirements.
- Data Governance:
- Quality Data Management: Ankercloud’s approach to data governance ensures that AI systems are trained on high-quality, non-biased data, meeting the AI Act’s standards.
- ISO/IEC 25024: This international standard outlines data quality measurement criteria like accuracy, completeness, consistency, and timeliness. Ankercloud's platform helps businesses assess these metrics to ensure their data meets regulatory expectations.
- Fairness and Bias Mitigation: According to the AI Act, data used for high-risk AI systems must be free from bias. Ankercloud incorporates AI fairness tools that detect and correct biased datasets, ensuring compliance with EU requirements for fairness, inclusivity, and non-discrimination.
- Traceability and Transparency: Ankercloud enables businesses to track and document the provenance of their data, ensuring compliance with the transparency demands of the AI Act. This feature helps maintain accountability and verifiability throughout the AI lifecycle.
- Data Security and Privacy: Ensuring that personal data is protected and privacy is maintained is crucial. Ankercloud helps implement security measures and privacy protocols compliant with the AI Act.
- ISO/IEC 27001: This standard defines the requirements for a comprehensive Information Security Management System (ISMS). Ankercloud helps businesses implement and manage information security strategies to ensure sensitive data is protected.
- GDPR (General Data Protection Regulation): GDPR is the key regulation in the EU for protecting personal data. Ankercloud supports businesses in meeting GDPR requirements, including data minimization, obtaining consent for data use, and ensuring data subject rights are respected.
- ISO/IEC 27701: This extension to ISO 27001 provides guidelines for privacy and helps implement a Privacy Information Management System (PIMS). Ankercloud incorporates this standard to assist businesses in complying with privacy regulations.
- Encryption and Access Controls: Ankercloud implements technologies such as end-to-end encryption and multi-factor authentication to ensure data is protected during transmission and storage. This helps businesses meet the AI Act's security requirements. Ankercloud’s comprehensive security and privacy solutions help businesses minimize the risk of data breaches and ensure compliance with relevant security standards, which is critical for using AI in line with the AI Act.
- Quality Data Management: Ankercloud’s approach to data governance ensures that AI systems are trained on high-quality, non-biased data, meeting the AI Act’s standards.
- Transparency and Documentation:
- Documentation Support: Ankercloud assists in creating and maintaining comprehensive documentation of AI system development and deployment, essential for audits and regulatory reviews.
- Human Oversight:
- Human-in-the-Loop Solutions: Ankercloud helps integrate human oversight mechanisms into AI systems, ensuring that critical decisions are reviewed by humans, as required by the AI Act.
- Training and Support:
- Ongoing Support: With continuous support and 24/7 account management delivered by a dedicated Managed Services team, Ankercloud continuously ensures that businesses stay compliant with evolving regulations and industry standards over the long term.
Conclusion
The EU’s AI Act marks a pivotal moment in the regulation of artificial intelligence, emphasizing the importance of ethical and responsible AI use. For businesses, navigating this new legal landscape may seem daunting. However, with Ankercloud’s comprehensive suite of solutions, companies can confidently manage compliance, mitigate risks, and leverage AI’s full potential within the bounds of the law. Partnering with Ankercloud ensures that your business not only meets regulatory requirements but also sets a benchmark for responsible AI innovation.
2
.jpg)
ALBeast Vulnerability: What It Is and How to Protect Your Applications
A newly identified vulnerability called "ALBeast" can cause a significant risk for AWS Application Load Balancer (ALB) using the load balancer authentication. This vulnerability was found by Miggo Research, meaning it is a severe problem that can lead to unauthorized access, data exfiltration, data breaches, and insider threats. Understanding it and mitigating this vulnerability is essential to organizations that are relying on the AWS Application Load Balancer to secure their applications.
Understanding ALBeast: What You Need to Know
ALBeast is a configuration-based vulnerability, the base of this vulnerability is "how AWS ALB handles the user authentication". ALB is a load balancer that operates on the OSI model's Layer 7. Its purpose is to handle the traffic by distributing the incoming application traffic across multiple targets like EC2 instances, containers, or IP addresses. On the one hand, the ALB improves reliability, fault tolerance, and scalability. And, the misconfiguration in ALB's authentication process can lead to a security breach where applications are exposed to the risk of being compromised.
The ALBeast vulnerability is critical because, with the help of this vulnerability, the attackers can bypass the critical security controls and lead to unauthorized access, by which attackers can access the applications without authentication. The Miggo research has identified over 15,000 potentially vulnerable applications out of the 371,000 ALBs analyzed, these potentially vulnerable applications do not contain the proper signer validation which is a key contributor to ALB-based authentication.
How Does ALBeast Vulnerability Work?
The ALBeast vulnerability exploits the weaknesses in how the applications validate the tokens provided by ALB. This vulnerability is raised because of two main issues which are:
- Missing Signer Validation: Many of the applications fail to verify the authenticity of the token signer, which means that the attacker can forge a token, manipulate it, impersonate it as a legitimate token, and present it to the application. Because of this misconfiguration, the application does not validate the signer's identity, accepts the token then grants the attacker unauthorized access.
- Misconfigured Security Groups: ALBeast vulnerability also takes advantage of misconfigurations in configured security groups that do not restrict traffic to trusted ALB instances. If an application accepts traffic from any source rather than limiting it to a specific ALB, an attacker can exploit this to bypass security controls.
Exploitation Scenario: How Attackers Exploit
- Setting Up a Malicious ALB: The attacker creates the malicious Application Load Balancer (ALB) which has similar configurations to the victim's setup.
- Forging a Token: The attacker forges a token and changes the information inside it, especially the part that says who issued it, to match what the victim’s application considers legitimate.
- Altering the Configurations: The attacker changes the configurations on the Malicious ALB so that AWS signs the token in a way that makes it look legitimate to the victim’s system.
- Bypassing Defenses: The attacker then uses this fake token to trick the victim’s application into bypassing security checks and gaining unauthorized access.
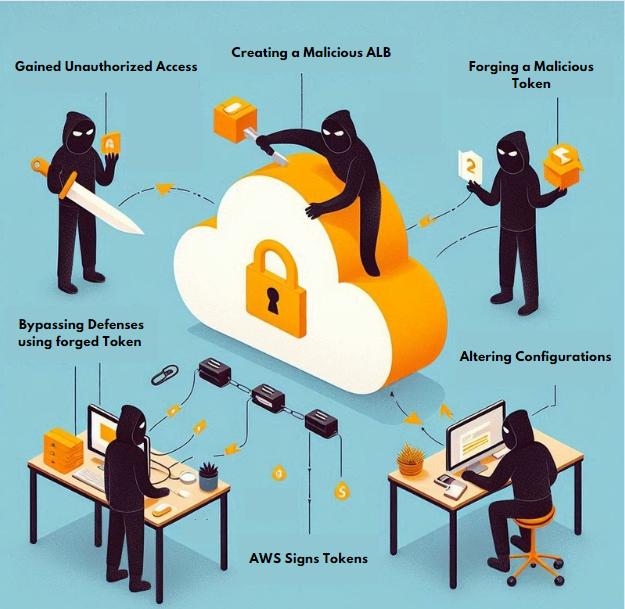
Best Practices to Mitigate ALBeast
- Verify Token Signer: Ensure that the applications are validating the signer of a JWT token provided with ALB to verify that the signer field from the JWT header matches the Amazon Resource Name (ARN) of the ALB signing the token.
- Restrict Traffic to Trusted ALBs: Configure your security groups to accept traffic only from trusted ALB instances. This can be achieved by referencing the ALB’s security group in the inbound rules for your target security group.
- Deploy Targets in Private Subnets: To prevent direct access from the public internet, deploy your ALB targets in private subnets without public IP addresses or Elastic IP addresses.
- Review and Update Configurations: Regularly review your application’s configurations to ensure they adhere to the latest AWS documentation. AWS has updated its authentication feature documentation to include new code for validating the signer, making it crucial for users to implement these changes.
Conclusion
The ALBeast vulnerability is a wake-up call for all organizations about how misconfigurations can lead to complex vulnerabilities in cloud-based applications. AWS has provided reliable tools for security management, though the responsibility to properly set up these tools is on the user. Organizations can immensely reduce the threat of unauthorized access to their applications based on the ALBeast issue by following the recommended mitigation strategies. These security risks accompany the continuous changes in cloud environments. The organizations should keep themselves up to date, ensure that they regularly update the configurations, and prepare to defend against threats like ALBeast.
2
Email Spoofing: What is it and How to Protect Against it
E-mail spoofing is a technique whereby the attacker forges the sender's address in such a way as to make the recipient believe it is coming from a trusted party. It could be a colleague in the office, a company, or even a financial institution. In this scenario, an attacker sends email headers in a manner such that the recipient would consider it to have come from a particular person, and the possibility of getting people to click on links they should not download attachments that will harm them or share with sensitive information becomes more probable.
Most of the current email systems lack a good feature that identifies forged sender addresses, and this explains why most phishing, spear-phishing, and business email compromise attacks rely on such a strategy.
For example, you might get an email that you are in both "To" and "From." Now, that doesn't mean someone has breached your email account. What they would do instead is pretend to be you or maybe another person you trust so that you open the email and may click on malicious links or release sensitive information.
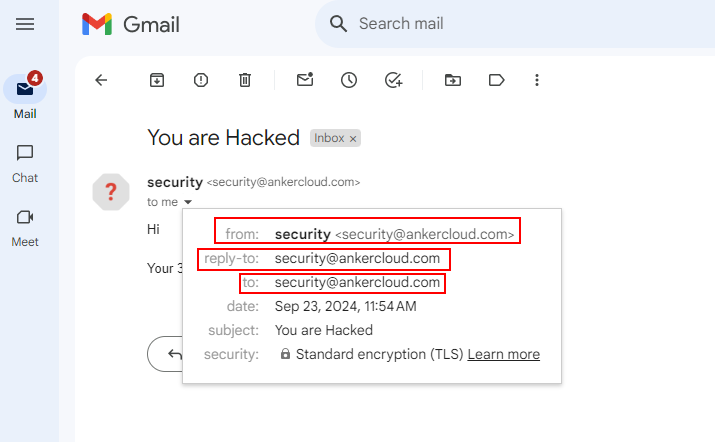
How to Identify a Spoofed Email?
Sent Folder does not have an e-mail - When you send yourself an e-mail, it always is going to be in your Sent folder. And consequently, since spammers spoofed your address, you'd never see it there because he sent it from nowhere, but it would appear as if it was from your account.


NOTE: If you compose an email to yourself and delete it from your sent folder, then you will no longer see the email in your inbox also.
Check the Original Email - Most E-mail systems will show you the "original" or "raw" version of an e-mail. This shows all the technical details of how the e-mail is sent. Look for a possible sign such as suspicious From, Reply-To, Return-Path, or Received, fields that don't look just right, maybe an indicator of a spoofed e-mail.
Grammar and spelling mistakes - Always keep an eye out for homoglyphs (Example: Ankercloud as Amk3rc10ud) and grammatical errors that a legitimate sender would never make.
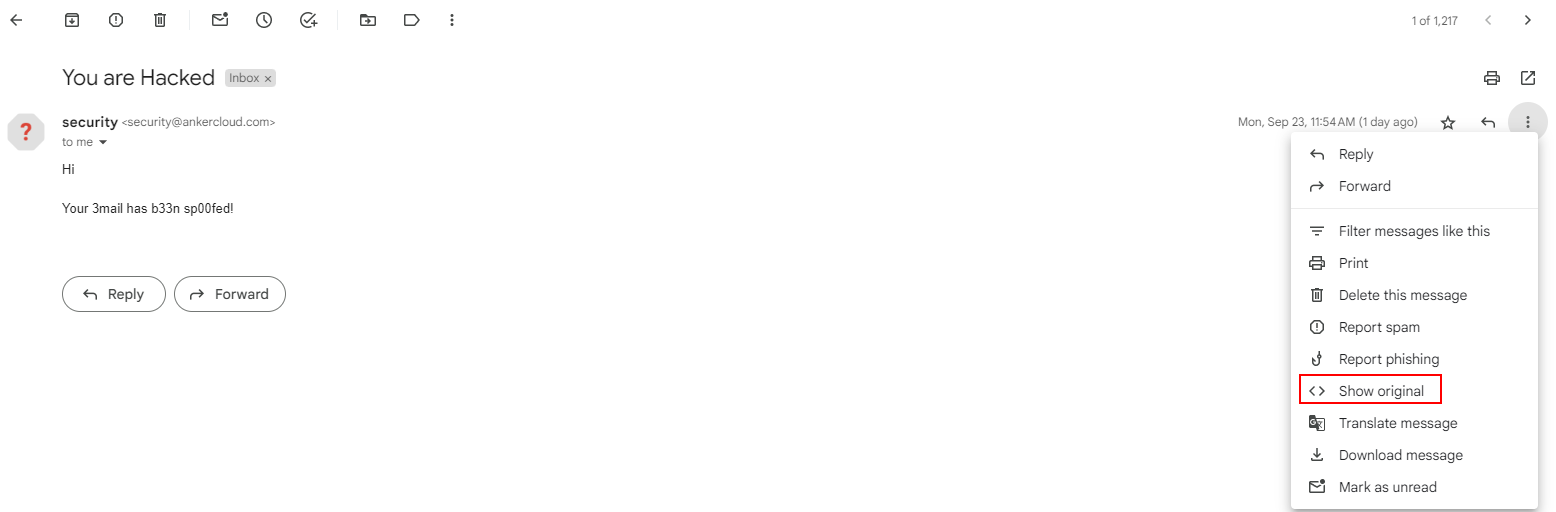
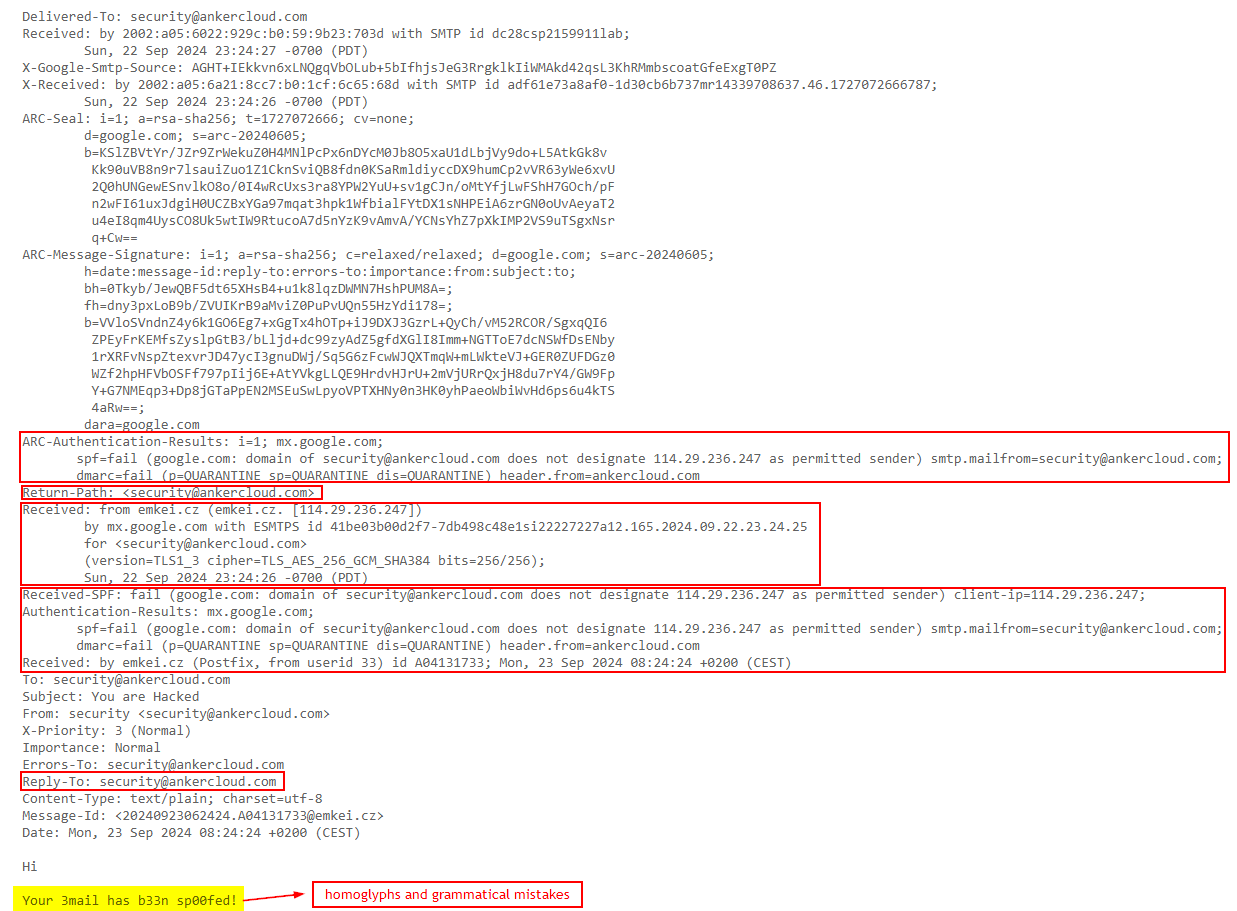
Preventive measures: protecting both yourself and your organization
- Implement the SPF, DKIM, and DMARC email security protocols as a layer of protection against spoofing which helps in verifying that an email was actually sent from the person that it says it is, if not then the email will go to spam/deleted immediately.
- Use spam filters- Generally, most email services have spam filters. These can filter suspicious-looking emails even before they reach your inbox. To be on the safe side, assume that your spam filter is active.
- Be Cautious - Be wary of opening any uncalled-for emails, particularly when suspicious, or asking for information that could be confidential.
What to Do If You Fall Victim to Spoofing Attack
- Don’t panic and disconnect the device from the internet immediately, to make sure no further damage is done by the malicious programs downloaded.
- Report the IT team on what happened so they can decide if sensitive information has been put at risk and make all the necessary arrangements to ensure safety measures are taken about your account.
- Change your passwords in case you think you got infected, change all the passwords right away from a different device, and implement/enforce 2FA for that added layer of security.
- Keep an eye on your email account and other accounts online for suspicious activities such as unauthorized login attempts or suspicious activities through your accounts.
Conclusion
Email spoofing is very hard to prevent, but definitely difficult to detect; if you are alert and think of some basic precautions, you should never fall for such a scam. So, always check suspicious emails and contact your IT/security team whenever in doubt, please ask for help.
Navigating the New AI Act in Europe: How Ankercloud can help your business to comply with it
In June 2023, the European Union took a significant step in regulating artificial intelligence with the introduction of the AI Act. This landmark legislation aims to create a legal framework for AI development and deployment across the EU, ensuring that AI technologies are used responsibly and ethically. For businesses leveraging AI, understanding and complying with the AI Act is crucial to avoid penalties and maintain consumer trust. This is where Ankercloud comes into play, offering solutions to help businesses navigate the complexities of the new law.
Understanding the AI Act
The AI Act classifies AI systems into three categories based on their potential risks:
- Unacceptable Risk: AI applications deemed a threat to safety, livelihoods, and rights are banned. This includes systems for social scoring by governments and real-time biometric identification in public spaces.
- High Risk: AI systems that impact critical areas such as healthcare, transport, and employment must meet strict requirements. This includes robust risk management, high-quality data, human oversight, and transparency.
- Limited Risk: Applications with a minimal risk, like chatbots, must ensure transparency and inform users that they are interacting with AI.
For businesses, especially those operating in high-risk categories, the AI Act mandates a comprehensive approach to risk management, data governance, and compliance documentation.
Challenges for Businesses
The introduction of the AI Act presents several challenges for businesses:
- Compliance Costs: Adhering to the stringent requirements of the AI Act may involve significant costs, particularly for SMEs.
- Technical Expertise: Ensuring that AI systems meet the law's standards requires specialized knowledge in AI development and data governance.
- Documentation and Transparency: Businesses need to maintain detailed records of their AI systems' design, testing, and deployment processes.
How Ankercloud Can Help
Ankercloud offers a suite of services designed to help businesses comply with the new AI Act seamlessly and efficiently. Here’s how Ankercloud can assist:
- Risk Assessment and Management:
- Comprehensive Risk Analysis: Ankercloud provides support to assess the risks associated with AI systems, helping businesses identify potential issues early in the development process in line with the industry best practices.
- Risk Mitigation Strategies: Through detailed analysis and expert guidance, Ankercloud helps implement robust risk mitigation strategies, ensuring compliance with the AI Act’s high-risk requirements.
- Data Governance:
- Quality Data Management: Ankercloud’s approach to data governance ensures that AI systems are trained on high-quality, non-biased data, meeting the AI Act’s standards.
- ISO/IEC 25024: This international standard outlines data quality measurement criteria like accuracy, completeness, consistency, and timeliness. Ankercloud's platform helps businesses assess these metrics to ensure their data meets regulatory expectations.
- Fairness and Bias Mitigation: According to the AI Act, data used for high-risk AI systems must be free from bias. Ankercloud incorporates AI fairness tools that detect and correct biased datasets, ensuring compliance with EU requirements for fairness, inclusivity, and non-discrimination.
- Traceability and Transparency: Ankercloud enables businesses to track and document the provenance of their data, ensuring compliance with the transparency demands of the AI Act. This feature helps maintain accountability and verifiability throughout the AI lifecycle.
- Data Security and Privacy: Ensuring that personal data is protected and privacy is maintained is crucial. Ankercloud helps implement security measures and privacy protocols compliant with the AI Act.
- ISO/IEC 27001: This standard defines the requirements for a comprehensive Information Security Management System (ISMS). Ankercloud helps businesses implement and manage information security strategies to ensure sensitive data is protected.
- GDPR (General Data Protection Regulation): GDPR is the key regulation in the EU for protecting personal data. Ankercloud supports businesses in meeting GDPR requirements, including data minimization, obtaining consent for data use, and ensuring data subject rights are respected.
- ISO/IEC 27701: This extension to ISO 27001 provides guidelines for privacy and helps implement a Privacy Information Management System (PIMS). Ankercloud incorporates this standard to assist businesses in complying with privacy regulations.
- Encryption and Access Controls: Ankercloud implements technologies such as end-to-end encryption and multi-factor authentication to ensure data is protected during transmission and storage. This helps businesses meet the AI Act's security requirements. Ankercloud’s comprehensive security and privacy solutions help businesses minimize the risk of data breaches and ensure compliance with relevant security standards, which is critical for using AI in line with the AI Act.
- Quality Data Management: Ankercloud’s approach to data governance ensures that AI systems are trained on high-quality, non-biased data, meeting the AI Act’s standards.
- Transparency and Documentation:
- Documentation Support: Ankercloud assists in creating and maintaining comprehensive documentation of AI system development and deployment, essential for audits and regulatory reviews.
- Human Oversight:
- Human-in-the-Loop Solutions: Ankercloud helps integrate human oversight mechanisms into AI systems, ensuring that critical decisions are reviewed by humans, as required by the AI Act.
- Training and Support:
- Ongoing Support: With continuous support and 24/7 account management delivered by a dedicated Managed Services team, Ankercloud continuously ensures that businesses stay compliant with evolving regulations and industry standards over the long term.
Conclusion
The EU’s AI Act marks a pivotal moment in the regulation of artificial intelligence, emphasizing the importance of ethical and responsible AI use. For businesses, navigating this new legal landscape may seem daunting. However, with Ankercloud’s comprehensive suite of solutions, companies can confidently manage compliance, mitigate risks, and leverage AI’s full potential within the bounds of the law. Partnering with Ankercloud ensures that your business not only meets regulatory requirements but also sets a benchmark for responsible AI innovation.
ALBeast Vulnerability: What It Is and How to Protect Your Applications
A newly identified vulnerability called "ALBeast" can cause a significant risk for AWS Application Load Balancer (ALB) using the load balancer authentication. This vulnerability was found by Miggo Research, meaning it is a severe problem that can lead to unauthorized access, data exfiltration, data breaches, and insider threats. Understanding it and mitigating this vulnerability is essential to organizations that are relying on the AWS Application Load Balancer to secure their applications.
Understanding ALBeast: What You Need to Know
ALBeast is a configuration-based vulnerability, the base of this vulnerability is "how AWS ALB handles the user authentication". ALB is a load balancer that operates on the OSI model's Layer 7. Its purpose is to handle the traffic by distributing the incoming application traffic across multiple targets like EC2 instances, containers, or IP addresses. On the one hand, the ALB improves reliability, fault tolerance, and scalability. And, the misconfiguration in ALB's authentication process can lead to a security breach where applications are exposed to the risk of being compromised.
The ALBeast vulnerability is critical because, with the help of this vulnerability, the attackers can bypass the critical security controls and lead to unauthorized access, by which attackers can access the applications without authentication. The Miggo research has identified over 15,000 potentially vulnerable applications out of the 371,000 ALBs analyzed, these potentially vulnerable applications do not contain the proper signer validation which is a key contributor to ALB-based authentication.
How Does ALBeast Vulnerability Work?
The ALBeast vulnerability exploits the weaknesses in how the applications validate the tokens provided by ALB. This vulnerability is raised because of two main issues which are:
- Missing Signer Validation: Many of the applications fail to verify the authenticity of the token signer, which means that the attacker can forge a token, manipulate it, impersonate it as a legitimate token, and present it to the application. Because of this misconfiguration, the application does not validate the signer's identity, accepts the token then grants the attacker unauthorized access.
- Misconfigured Security Groups: ALBeast vulnerability also takes advantage of misconfigurations in configured security groups that do not restrict traffic to trusted ALB instances. If an application accepts traffic from any source rather than limiting it to a specific ALB, an attacker can exploit this to bypass security controls.
Exploitation Scenario: How Attackers Exploit
- Setting Up a Malicious ALB: The attacker creates the malicious Application Load Balancer (ALB) which has similar configurations to the victim's setup.
- Forging a Token: The attacker forges a token and changes the information inside it, especially the part that says who issued it, to match what the victim’s application considers legitimate.
- Altering the Configurations: The attacker changes the configurations on the Malicious ALB so that AWS signs the token in a way that makes it look legitimate to the victim’s system.
- Bypassing Defenses: The attacker then uses this fake token to trick the victim’s application into bypassing security checks and gaining unauthorized access.
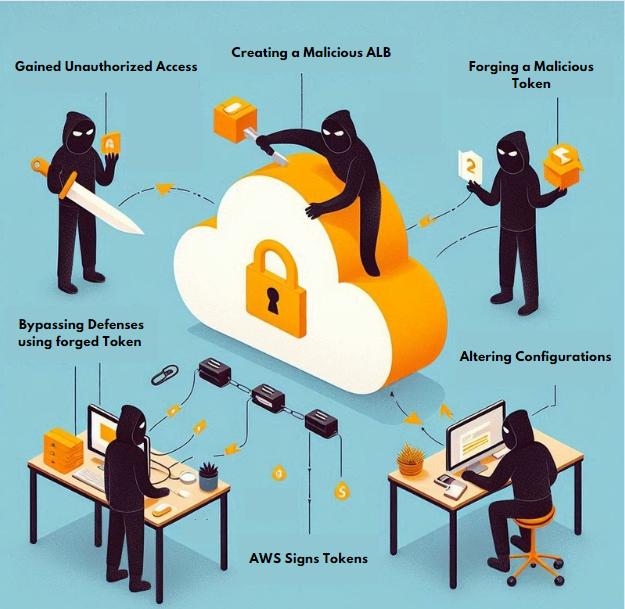
Best Practices to Mitigate ALBeast
- Verify Token Signer: Ensure that the applications are validating the signer of a JWT token provided with ALB to verify that the signer field from the JWT header matches the Amazon Resource Name (ARN) of the ALB signing the token.
- Restrict Traffic to Trusted ALBs: Configure your security groups to accept traffic only from trusted ALB instances. This can be achieved by referencing the ALB’s security group in the inbound rules for your target security group.
- Deploy Targets in Private Subnets: To prevent direct access from the public internet, deploy your ALB targets in private subnets without public IP addresses or Elastic IP addresses.
- Review and Update Configurations: Regularly review your application’s configurations to ensure they adhere to the latest AWS documentation. AWS has updated its authentication feature documentation to include new code for validating the signer, making it crucial for users to implement these changes.
Conclusion
The ALBeast vulnerability is a wake-up call for all organizations about how misconfigurations can lead to complex vulnerabilities in cloud-based applications. AWS has provided reliable tools for security management, though the responsibility to properly set up these tools is on the user. Organizations can immensely reduce the threat of unauthorized access to their applications based on the ALBeast issue by following the recommended mitigation strategies. These security risks accompany the continuous changes in cloud environments. The organizations should keep themselves up to date, ensure that they regularly update the configurations, and prepare to defend against threats like ALBeast.
FAQs
Some benefits of using cloud computing services include cost savings, scalability, flexibility, reliability, and increased collaboration.
Ankercloud takes data privacy and compliance seriously and adheres to industry best practices and standards to protect customer data. This includes implementing strong encryption, access controls, regular security audits, and compliance certifications such as ISO 27001, GDPR, and HIPAA, depending on the specific requirements of the customer. Learn More
The main types of cloud computing models are Infrastructure as a Service (IaaS), Platform as a Service (PaaS), and Software as a Service (SaaS). Each offers different levels of control and management for users.
Public clouds are owned and operated by third-party providers, private clouds are dedicated to a single organization, and hybrid clouds combine elements of both public and private clouds. The choice depends on factors like security requirements, scalability needs, and budget constraints.
Cloud computing services typically offer pay-as-you-go or subscription-based pricing models, where users only pay for the resources they consume. Prices may vary based on factors like usage, storage, data transfer, and additional features.
The process of migrating applications to the cloud depends on various factors, including the complexity of the application, the chosen cloud provider, and the desired deployment model. It typically involves assessing your current environment, selecting the appropriate cloud services, planning the migration strategy, testing and validating the migration, and finally, executing the migration with minimal downtime.
Ankercloud provides various levels of support to its customers, including technical support, account management, training, and documentation. Customers can access support through various channels such as email, phone, chat, and a self-service knowledge base.
The Ankercloud Team loves to listen
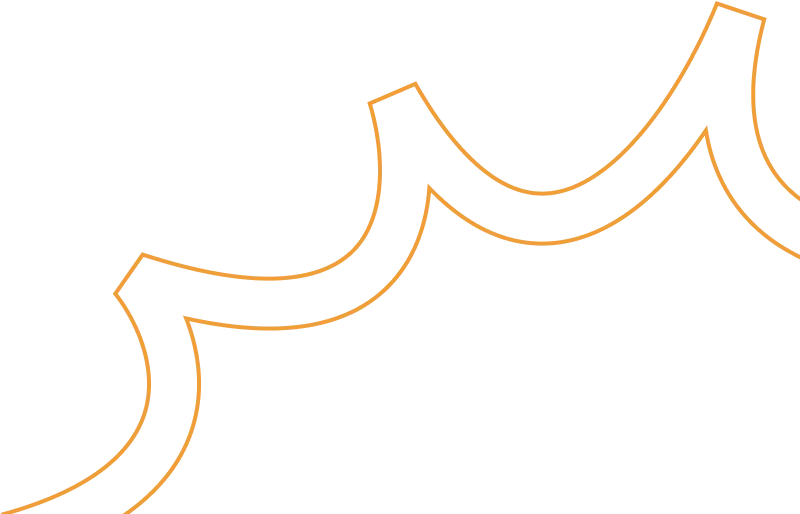
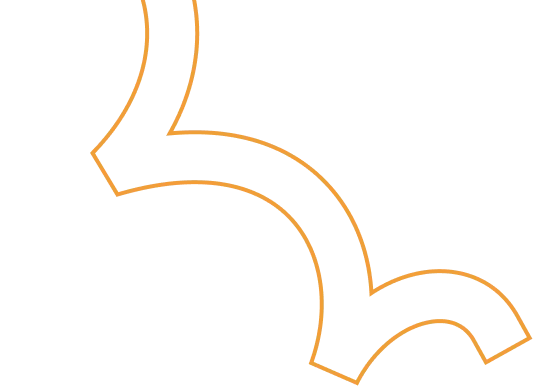