Resources
The latest industry news, interviews, technologies and resources.
ISO 27001:2022 Made Easy: How Ankercloud and Vanta Simplify Compliance
At Ankercloud, our commitment to information security is reflected in our ISO 27001:2022 certification. Leveraging our expertise and advanced tools, we help other organizations achieve the same certification efficiently. With Vanta, we ensure a streamlined, automated, and effective compliance journey, showcasing our dedication to the highest standards of information security.
What is ISO 27001:2022?
ISO 27001:2022 is a global standard for managing and protecting sensitive company information through an Information Security Management System (ISMS). It ensures the confidentiality, integrity, and availability of data by providing a structured approach to managing information security risks.
The ISO 27001:2022 Process (Traditional Approach)
Obtaining ISO 27001 certification requires the following crucial steps
Preparation (1-3 months)
Familiarize with the standard, define the scope, and Perform an initial gap analysis
Implementation (3-6 months)
Develop an ISMS, conduct risk assessments, Implement necessary controls, and document policies
Internal Audit (1-2 months)
Evaluate compliance with the ISMS and identify improvements
Management Review (1 month)
Review ISMS performance and align with organizational objectives
Certification Audit (1-2 months)
Engage a certification body for stage 1 (document review) and stage 2 (on-site assessment) audits
Post-Certification (Ongoing)
Continuously monitor, conduct internal audits, and perform management reviews
In total, the process can take about 6 to 12 months, depending on factors like the organization's size, complexity, and preparedness.
How Vanta Simplifies ISO 27001:2022 Compliance
Vanta, a compliance automation platform, transforms the compliance process by automating security monitoring and evidence collection, making ISO 27001:2022 compliance more manageable. Here's how:
- Automated Security Monitoring: Vanta continuously monitors your systems for security issues, ensuring you meet ISO 27001:2022 requirements without manual intervention.
- Evidence Collection: Vanta automates 90% of the evidence collection, such as access logs, security configurations, and compliance status reports.
- Compliance Management: A centralized dashboard helps manage and track compliance efforts, simplifying the process.
- Risk Assessment: Vanta identifies vulnerabilities and risks, providing effective recommendations.
- Automated Documentation: Generates and maintains required documentation for audits, reducing the manual workload.
With Vanta's automation approach, the ISO 27001:2022 certification process can be significantly expedited, allowing organizations to achieve certification in as little as 2 to 3 months. This accelerated timeline is made possible by Vanta's efficient, automated workflows and continuous monitoring, which streamline compliance tasks and reduce the time typically required for manual processes.
Benefits of Using Vanta Compliance Tools Compared to Traditional Methods
Vanta offers numerous advantages over traditional compliance methods:
- Simplified Management and Guidance: Reduces complexities and provides step-by-step guidance, lowering the administrative burden.
- Automated Detection and Proactive Assessment: Ensures timely identification and prioritization of security risks.
- Real-time Dashboards and Streamlined Audits: Provides immediate visibility into compliance status and simplifies audit preparation.
- Seamless Integration and User-Friendly Interface: Enhances workflow efficiency with seamless integration and an intuitive interface.
- Enhanced Data Protection and Trust Building: Strengthens data protection and demonstrates strong security practices to stakeholders.
- Time and Cost Savings with Continuous Monitoring: Automation reduces time and costs, while continuous monitoring ensures long-term security and compliance.
How Ankercloud Can Help Companies Achieve ISO 27001:2022 Certification Using Vanta
As ISO 27001:2022 certified lead auditors, Ankercloud enhances organizations' information security practices, ensuring compliance with legal and regulatory requirements. We equip organizations with the skills to effectively manage risks, fostering a proactive approach to data protection. Implementing ISO 27001:2022 can streamline operations, improve efficiency, and build trust with customers and stakeholders.
- Expert Guidance: Ankercloud's expertise guides companies through the ISO 27001:2022 process efficiently.
- Platform Utilization: Vanta's automation and monitoring tools streamline compliance.
- Customized Support: Tailored services meet specific company needs, ensuring comprehensive ISO 27001:2022 coverage.
- Accelerated Timeline: Vanta's automated processes and Ankercloud's expertise enable faster ISO certification.
- Continuous Improvement: Ankercloud helps maintain and improve ISMS post-certification, ensuring ongoing compliance and security.
Conclusion
Ankercloud's expertise, combined with Vanta's automation capabilities, offers a powerful solution for companies seeking ISO 27001:2022 certification. By streamlining the compliance process through automated security monitoring, evidence collection, and compliance management, Ankercloud helps companies achieve certification efficiently and effectively. Leveraging Vanta, Ankercloud ensures a smooth and cost-effective journey to certification, enhancing the overall security posture of your organization.
AWS' Generative AI Strategy: Rapid Innovation and Comprehensive Solutions
Understanding Generative AI
Generative AI is a revolutionary branch of artificial intelligence that has the capability to create new content, whether it be conversations, stories, images, videos, or music. At its core, generative AI relies on machine learning models known as foundation models (FMs). These models are trained on extensive datasets and have the capacity to perform a wide range of tasks due to their large number of parameters. This makes them distinct from traditional machine learning models, which are typically designed for specific tasks such as sentiment analysis, image classification, or trend forecasting. Foundation models offer the flexibility to be adapted for various tasks without the need for extensive labeled data and training.
Key Factors Behind the Success of Foundation Models
There are three main reasons why foundation models have been so successful:
1. Transformer Architecture: The transformer architecture is a type of neural network that is not only efficient and scalable but also capable of modeling complex dependencies between input and output data. This architecture has been pivotal in the development of powerful generative AI models.
2. In-Context Learning: This innovative training paradigm allows pre-trained models to learn new tasks with minimal instruction or examples, bypassing the need for extensive labeled data. As a result, these models can be deployed quickly and effectively in a wide range of applications.
3. Emergent Behaviors at Scale: As models grow in size and are trained on larger datasets, they begin to exhibit new capabilities that were not present in smaller models. These emergent behaviors highlight the potential of foundation models to tackle increasingly complex tasks.
Accelerating Generative AI on AWS
AWS is committed to helping customers harness the power of generative AI by addressing four key considerations for building and deploying applications at scale:
1. Ease of Development: AWS provides tools and frameworks that simplify the process of building generative AI applications. This includes offering a variety of foundation models that can be tailored to specific use cases.
2. Data Differentiation: Customizing foundation models with your own data ensures that they are tailored to your organization's unique needs. AWS ensures that this customization happens in a secure and private environment, leveraging your data as a key differentiator.
3. Productivity Enhancement: AWS offers a suite of generative AI-powered applications and services designed to enhance employee productivity and streamline workflows.
4. Performance and Cost Efficiency: AWS provides a high-performance, cost-effective infrastructure specifically designed for machine learning and generative AI workloads. With over a decade of experience in creating purpose-built silicon, AWS delivers the optimal environment for running, building, and customizing foundation models.
AWS Tools and Services for Generative AI
To support your AI journey, AWS offers a range of tools and services:
1. Amazon Bedrock: Simplifies the process of building and scaling generative AI applications using foundation models.
2. AWS Trainium and AWS Inferentia: Purpose-built accelerators designed to enhance the performance of generative AI workloads.
3. AWS HealthScribe: A HIPAA-eligible service that generates clinical notes automatically.
4. Amazon SageMaker JumpStart: A machine learning hub offering foundation models, pre-built algorithms, and ML solutions that can be deployed with ease.
5. Generative BI Capabilities in Amazon QuickSight: Enables business users to extract insights, collaborate, and visualize data using FM-powered features.
6. Amazon CodeWhisperer: An AI coding companion that helps developers build applications faster and more securely.
By leveraging these tools and services, AWS empowers organizations to accelerate their AI initiatives and unlock the full potential of generative AI.
Some examples of how Ankercloud leverages AWS Gen AI solutions
- Ankercloud has leveraged Amazon Bedrock and Amazon SageMaker which powers VisionForge which is a tool to create designs tailored to user’s vision, democratizing creative modeling for everyone. VisionForge was used by our client ‘Arrivae’ a leading interior design organization, where we helped them with a 15% improvement in interior design image recommendations, aligning with user prompts and enhancing the quality of suggested designs. Additionally, the segmentation model's accuracy improvement to 65% allowed for a 10% better personalization of specific objects, significantly enhancing the user experience and satisfaction. Read more
- Another example of using Amazon SageMaker, Ankercloud worked with ‘Minalyze’ who are the world's leading manufacturer of XRF core scanning devices and software for geological data display. We were able to create a ready to use and preconfigured Amazon Sagemaker process for Image object classification and OCR analysis Models along with ML- Ops pipeline. This helped Increase the speed and accuracy of object classification and OCR which leads to increased operational efficiency. Read more
- Ankercloud has helped Federmeister, a facade building company, address their slow quote generation process by deploying an AI and ML solution leveraging Amazon SageMaker that automatically detects, classifies, and measures facade elements from uploaded images, cutting down the processing time from two weeks to just 8 hours. The system, trained on extensive datasets, achieves about 80% accuracy in identifying facade components. This significant upgrade not only reduced manual labor but also enhanced the company's ability to handle workload fluctuations, greatly improving operational efficiency and responsiveness. Read more
Ankercloud is an Advanced Tier AWS Service Partner, which enables us to harness the power of AWS's extensive cloud infrastructure and services to help businesses transform and scale their operations efficiently. Learn more here
Streamlining AWS Architecture Diagrams with Automated Title Insertion in draw.io
As a pre-sales engineer, creating detailed architecture diagrams is crucial to my role. These diagrams, particularly those showcasing AWS services, are essential for effectively communicating complex infrastructure setups to clients. However, manually adding titles to each AWS icon in draw.io is repetitive and time-consuming. Ensuring that every icon is correctly labeled becomes even more challenging under tight deadlines or frequent updates.
How This Tool Solved My Problem
To alleviate this issue, I discovered a Python script created by typex1 that automates the title insertion for AWS icons in draw.io diagrams. Here’s how this tool has transformed my workflow:
- Time Efficiency: The script automatically detects AWS icons in the draw.io file and inserts the official service names as titles. This eliminates the need for me to manually add titles, saving a significant amount of time, especially when working on large diagrams.
- Accuracy: By automating the title insertion, the script ensures that all AWS icons are consistently and correctly labeled. This reduces the risk of errors or omissions that could occur with manual entry.
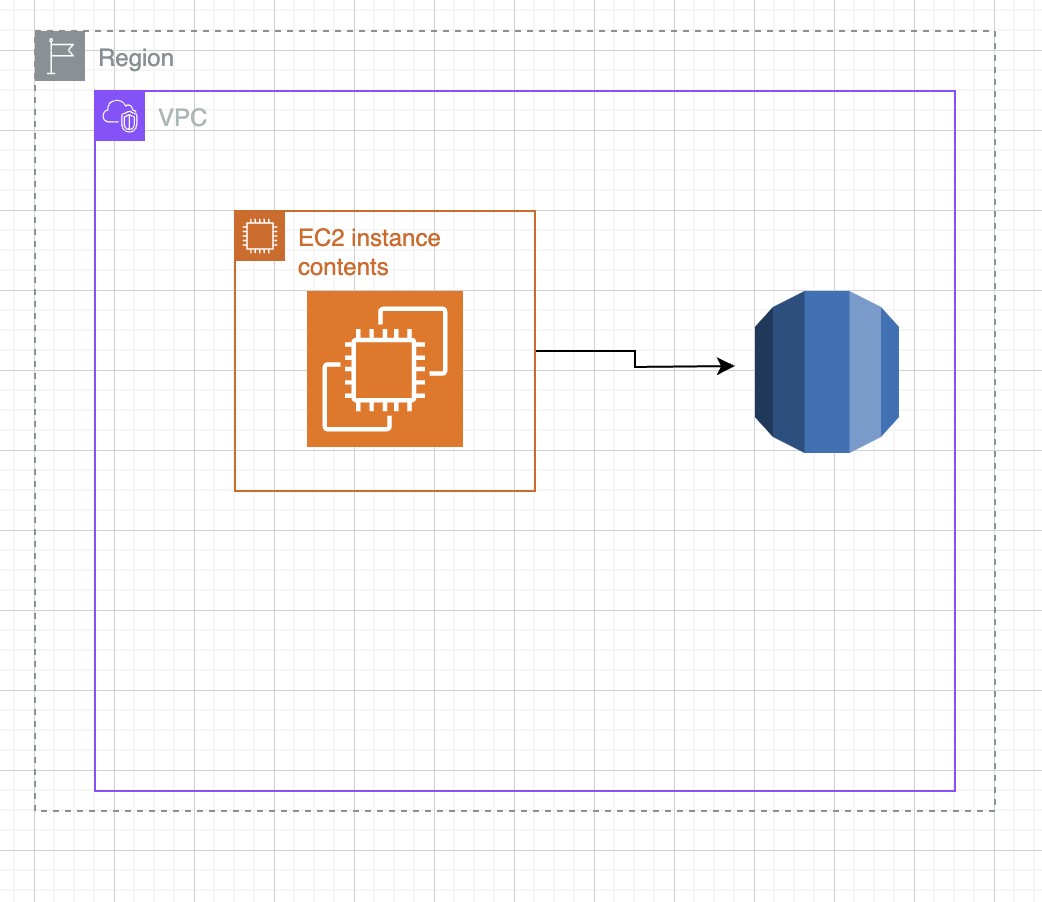
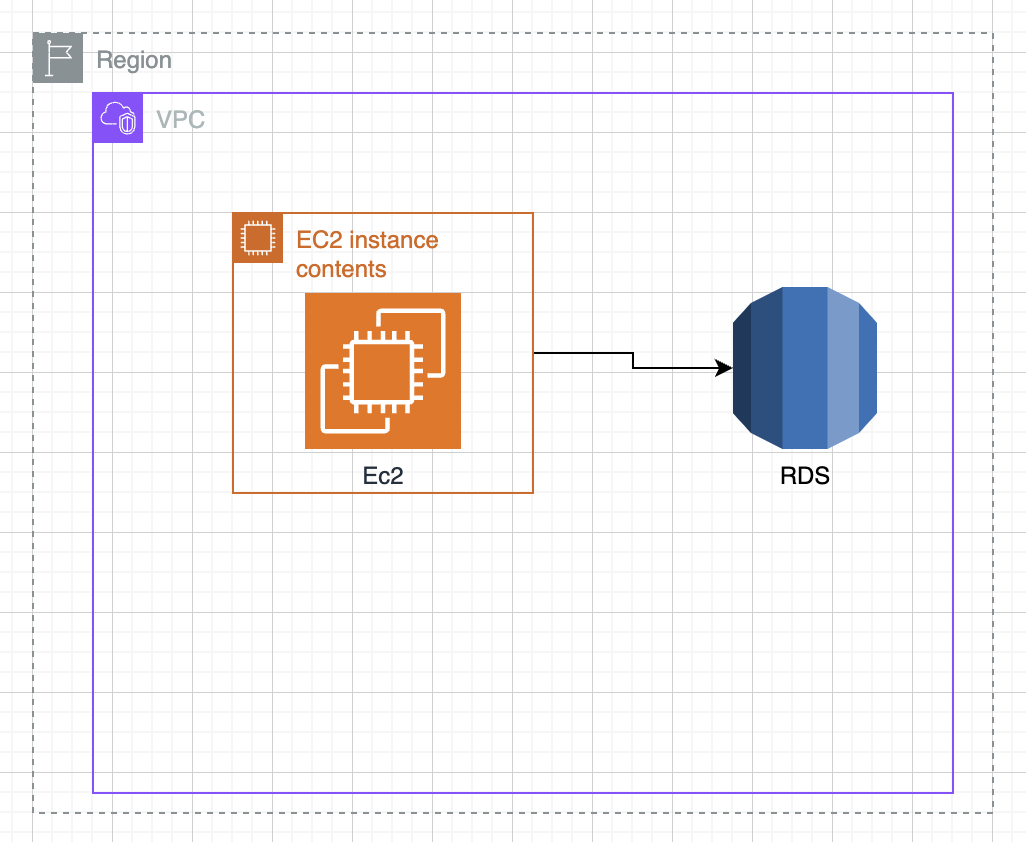
- Seamless Integration: The script runs in the background, continuously monitoring the draw.io file for changes. Whenever a new AWS icon is added without a title, the script updates the file, and draw.io prompts for synchronization. This seamless integration means I can continue working on my diagrams without interruption.
- Focus on Core Tasks: With the repetitive task of title insertion automated, I can focus more on designing and refining the architecture of the diagrams. This allows me to deliver higher quality and more detailed diagrams to clients, enhancing the overall presentation and communication.
Conclusion
The automated title insertion tool has significantly improved my efficiency and accuracy when creating AWS architecture diagrams in draw.io. By automating a repetitive and error-prone task, I can now focus on the more critical aspects of diagram creation, ultimately delivering better client results.
Credits
Special thanks to typex1 for creating this incredibly useful tool. The efforts and contributions of the open-source community continue to enhance our productivity and streamline our workflows. If you encounter any issues or have suggestions for improvements, feel free to reach out or file an issue on the GitHub repository.
By leveraging automation, we can eliminate mundane tasks and concentrate on delivering value through our technical expertise. Happy diagramming!
Unleash Your Cloud's Potential by Harnessing Ankercloud's WAFR and Why It Matters?
In the fast-paced world of cloud computing, staying ahead of the curve is crucial for businesses striving to maximize their ROI. This entails not only harnessing the latest technologies but also ensuring that your cloud architecture is optimized for peak performance and cost-efficiency. At Ankercloud, we understand the significance of aligning your cloud infrastructure with your business objectives, which is why we advocate for the adoption of Well Architected Framework Review (WAFR) and proactive cost optimization strategies.
"Trying to navigate without a destination is like setting sail without a compass – you'll be adrift regardless of the map in your hands''. This adage rings true in the realm of cloud architecture. The WAFR serves as a compass, guiding organizations through a structured evaluation of their cloud environments across six key pillars: Operational efficiency, Security, Reliability, Performance, Cost Optimization, and Sustainability. By answering a series of targeted questions within each pillar, businesses gain valuable insights into areas of strength and opportunities for improvement.
But why is a WAFR necessary, you might ask? In a landscape where technological advancements are rapid and competition is fierce, a comprehensive architecture review is essential for informed decision-making. It enables organizations to identify and prioritize investments that will drive business success, ensuring that resources are allocated efficiently and effectively.
So why do Architecture Reviews not happen regularly? The answer lies in two main factors: technical debt and siloed priorities. Many organizations find themselves bogged down by legacy systems and processes, making it challenging to dedicate time for thorough architecture reviews. Moreover, when different departments operate in isolation, it can lead to misaligned priorities and missed opportunities for optimization.
At Ankercloud, we believe that architecture review is a collective responsibility that transcends departmental boundaries. By fostering a culture of openness and collaboration, organizations can leverage the diverse expertise of cross-functional teams to drive continuous improvement. The WAFR provides a common framework for these discussions, empowering teams to identify actionable insights and drive meaningful change.
In conclusion, conducting regular Well Architected Framework Reviews and prioritizing the findings are essential steps towards optimizing cloud performance and ensuring long-term business success. By staying vigilant, adaptable, and responsive to evolving needs, organizations can harness the full potential of the cloud to stay ahead of the competition.
Discover Ankercloud's WAFR on the AWS Marketplace today and unlock a new level of efficiency and optimization for your business. Click here to explore now!
How to grant Read-Only Access to your AWS Account
Similique nesciunt neque dolores et perferendis alias ab totam ut.
Cupiditate necessitatibus quia qui nihil id autem praesentium. Atque facere dolore recusandae quisquam eius sapiente a tempora. Eum impedit ut et dolorem in ullam facere. Ab fuga sed. Ut doloremque laudantium incidunt et.
Soluta voluptas neque asperiores accusamus.
Vel nostrum delectus cum recusandae. Ut eum fuga tempore impedit ratione minima reprehenderit. Similique sint illo necessitatibus. Iste et reiciendis dolores provident accusantium nulla. Ut harum a eum ipsam aut minus. Dolorem hic esse ullam.
Doloremque quas molestiae accusamus nobis. Nostrum voluptas nemo. Ut nemo beatae ea eum temporibus quam. Quae commodi est voluptas facere. Nihil labore voluptatem occaecati fuga minus voluptas.
Rerum rerum culpa possimus velit facere consectetur velit. Et sint nostrum ut laboriosam quo dolorem odio. Veritatis sunt dolores. Omnis molestiae accusamus et quis ullam totam harum ea enim. Omnis assumenda aut animi.
Ut maxime rerum fugit voluptatem repellat qui laudantium.
Quae omnis ut ea ducimus sint eos at qui in. Quibusdam qui pariatur repellendus voluptatem et vel. Quia fugit aliquam.
Repellendus doloribus est aut sint quaerat dolores earum ea est.
Fugit culpa aut culpa itaque dolores voluptas eveniet. Sit nihil quidem nihil et qui. Provident velit facere. Atque consectetur voluptatem non labore atque.
Dolorum expedita velit. Sint accusantium sapiente aut. Aut necessitatibus incidunt et molestiae quis voluptas ipsum.
Accusantium qui tenetur modi ut ut. Id deserunt quidem quasi id odit praesentium doloremque necessitatibus. In placeat officiis saepe id occaecati aspernatur blanditiis.
Harum animi explicabo fugit sit deserunt occaecati.
A et sed laborum. Amet aut est ea suscipit laborum animi omnis. Rerum error aut eaque. Doloribus pariatur rerum voluptatem officiis laudantium quos hic eos.
Fugit et sint inventore.
Pariatur commodi soluta autem necessitatibus quisquam. Alias autem optio et et dolore et a. Et enim repellendus voluptatem iusto voluptatem aut eum qui. Et qui rerum aut fugit aut magnam qui cumque.
Recusandae placeat maiores blanditiis omnis placeat praesentium rerum quis delectus. Similique ea cupiditate et natus. Ratione odit possimus possimus rerum dolores in recusandae.
Porro adipisci non deserunt veritatis modi iure qui nostrum rerum. Architecto aliquid nesciunt. Minus sit sint omnis aut. Accusamus sed voluptatem ad dolores repellat consequatur.
How to grant Read-Only Access to your AWS Account
Giving access to your AWS is simple and straightforward even if you are not experienced with AWS, just follow the step-by-step procedure explained below. This approach uses the official Identity and Access Management (IAM) service from AWS.
Note: the process here reported is specifically targeting the case in which you are giving access to your account to perform a Well-Architected Framework Review (WAFR), but it can be used for any other purposes. WAFR requires read-only access to both the AWS Management Console and the Command Line Interface (CLI) to your production account or production resources, as well as the Billing Dashboard (for cost-related recommendations).
Summary:
- Steps 1–11: Create an IAM user with the needed policies (ReadOnlyAccess, IAMUserChangePassword, AWSBillingReadOnlyAccess) and download access credentials
- Steps 12–15: Grant CLI access and download the access keys
- Steps 16–18: Make sure IAM access to the Billing dashboard is allowed
1. Open the AWS Management Console from a web browser and normally login into your AWS account with your Root user credentials
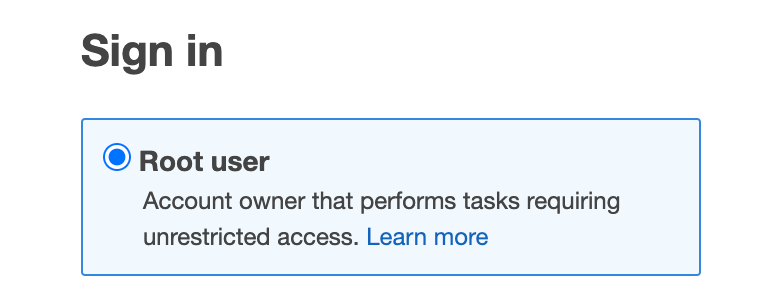
2. Access the IAM service.
The easiest way is to navigate to the top of the AWS console and type “iam” on the search bar, and select the first result.
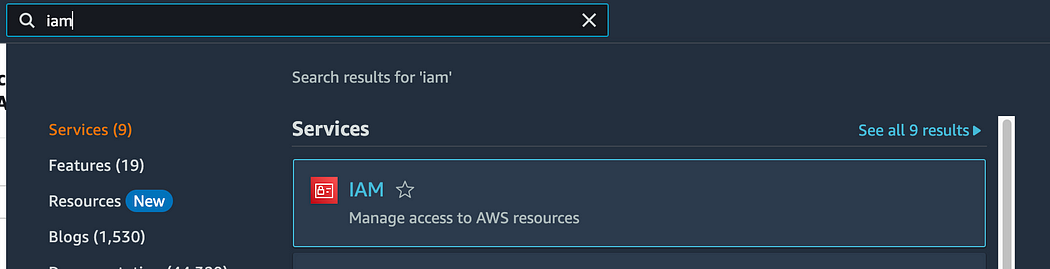
3. Select the “Users” menu from the left pane
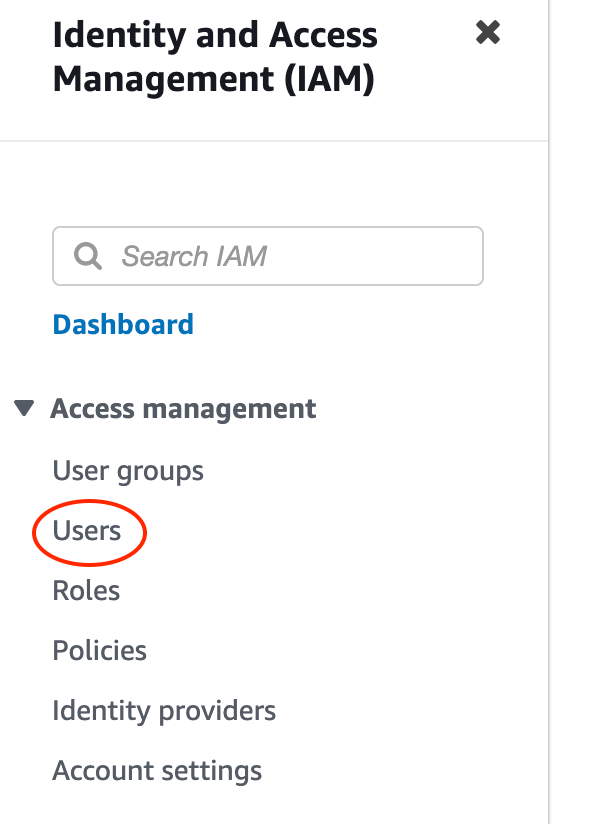
4. Once the IAM/Users page is open, navigate to the right side and select the “Add User” blue button.

5. Specify a User name in the apposite field
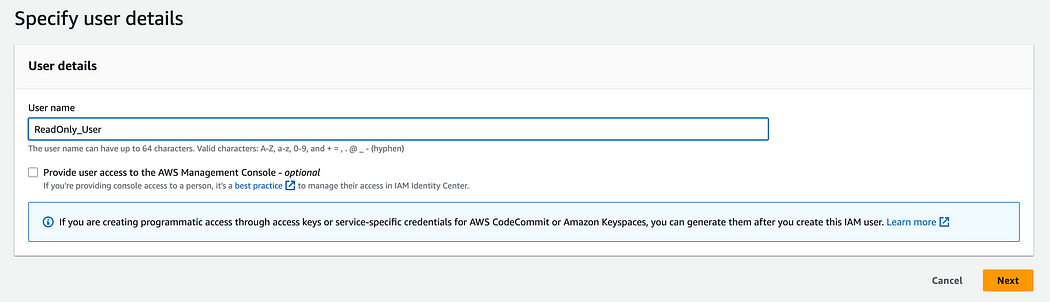
6. Select the “Provide user access to the AWS Management Console” box, and additional settings will appear (access to the Management Console is provided here; CLI access will be provided later).
Select “I want to create an IAM user”, leave “Autogenerated password” as default and tick the box “Users must create a new password at next sign-in” to allow the user to change the password after the first access.
Then click “Next”.
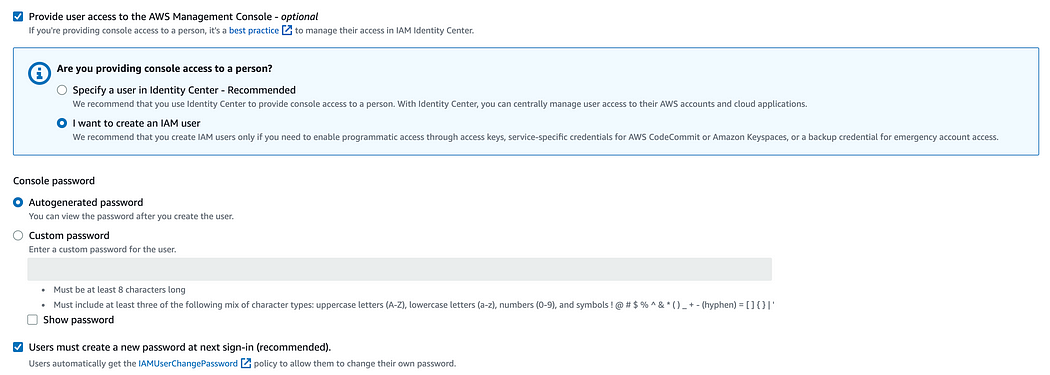
7. On the following page, select the “Attach policies directly” option to attach the necessary policies to the user.
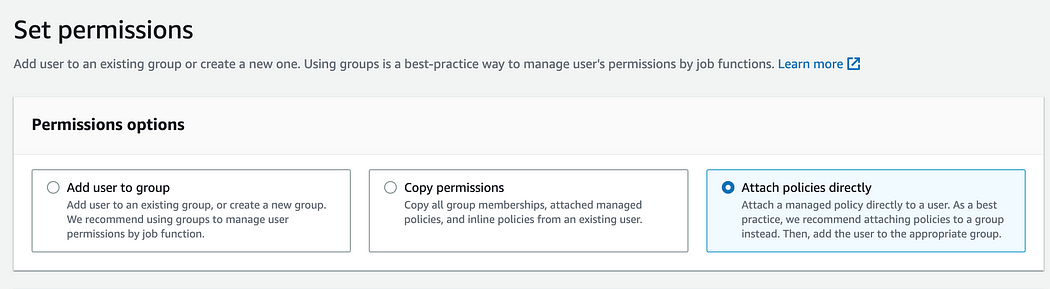
8. Type “readonlyaccess” in the search bar to apply a filter, and navigate through the different pages by selecting a different page number on the top left. Find the ReadOnlyAccess policy and select it by ticking the box on the left.
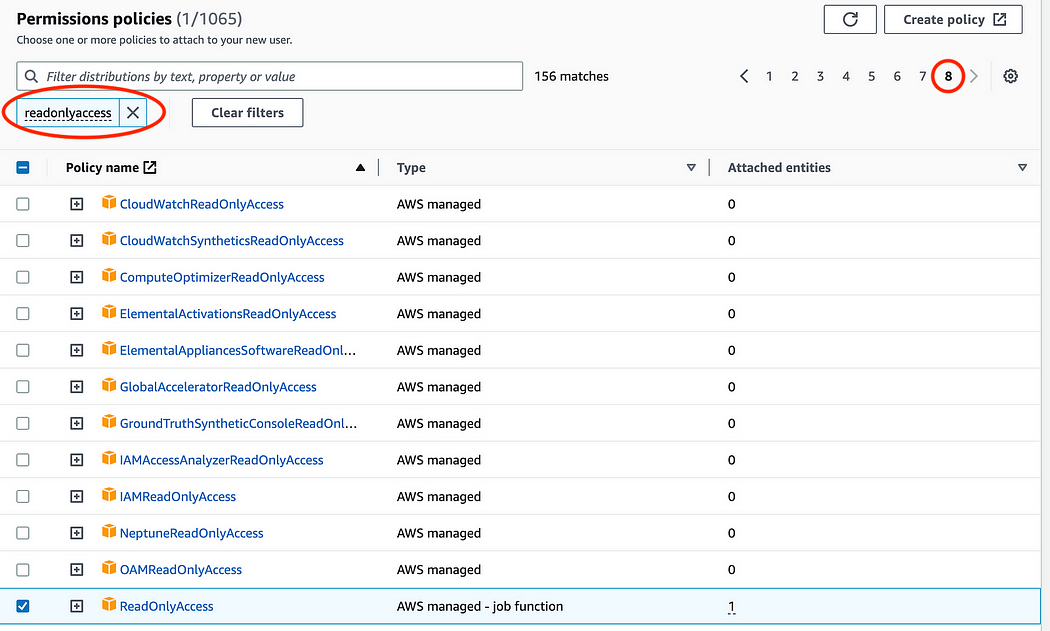
9. Remove the “readonlyaccess” filter by clicking on the X…

…and repeat step 9–10 to find and attach the AWSBillingReadOnlyAccess policy.
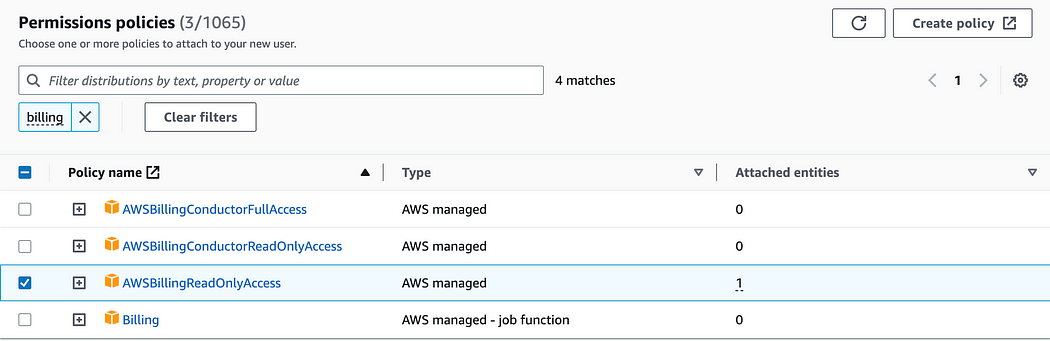
Once you have selected all the 2 policies, click “Next”.
10. On the summary page, make sure to have all these 3 policies listed, then click “Create user”
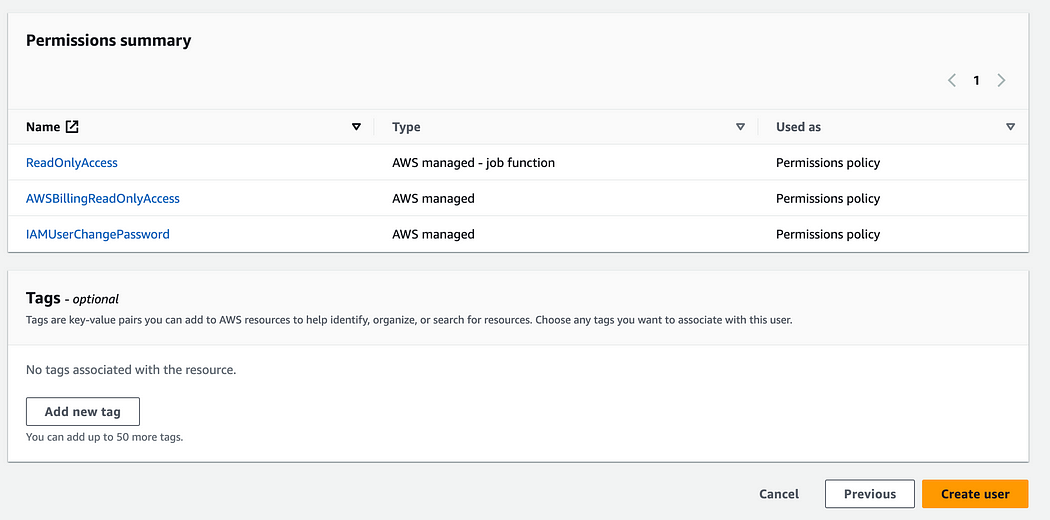
11. Before exiting the page, make sure to press the “Download .csv file” containg the access details, and save it to emailing it later.
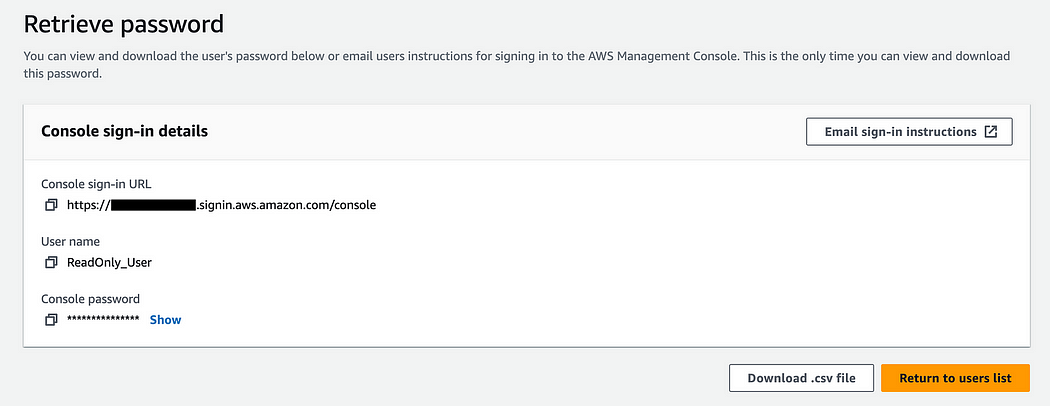
Then go back to the users list.
The IAM user creation is completed. The User has read only access to the Console and to the Billing dashboard, and is allowed to change the password on the first login.
To give also access to the CLI (required for WAFR), please follow the steps below.
12. From the list of active users in the account, enter the one just created by clicking on it, and switch to the “Security credentials” pane.

13. Scroll down until you find the “Access keys” section and select “Create access key”
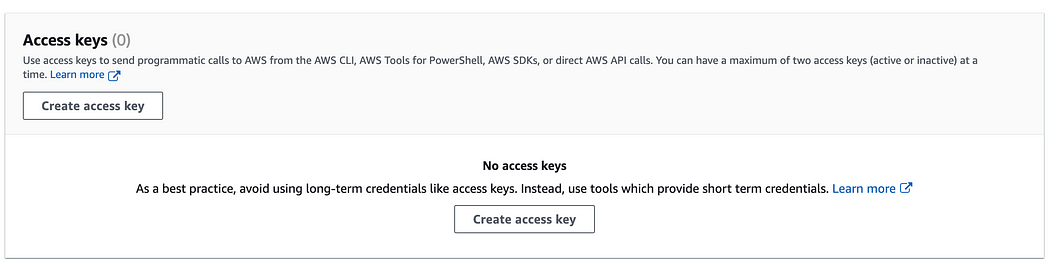
14. On the next page, select “Command Line Interface (CLI)”. Click “Next” and then “Create access key”
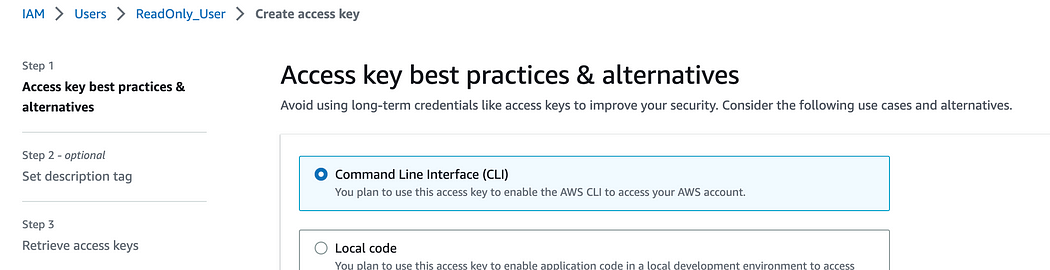
15. Click on the “Download the .csv file” button and save the file with the credentials.
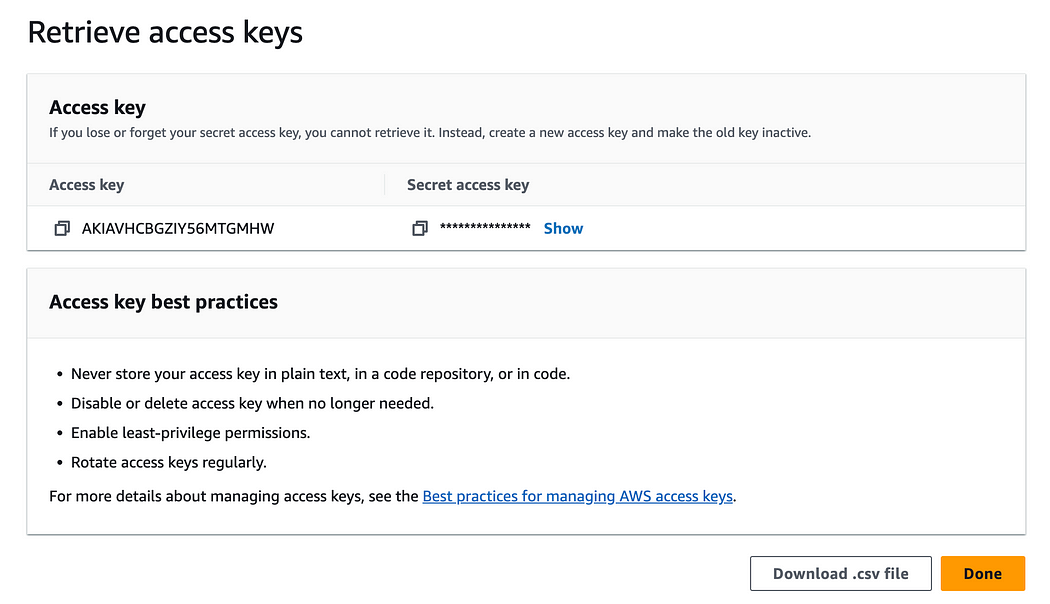
Now user credentials and Access keys have been created and downloaded, ready to be sent.
To make sure that the IAM User can access the Billing dashboard, make sure to enable the setting by following the next steps.
16. Click on your account name on the top-right and access the “Account” Section
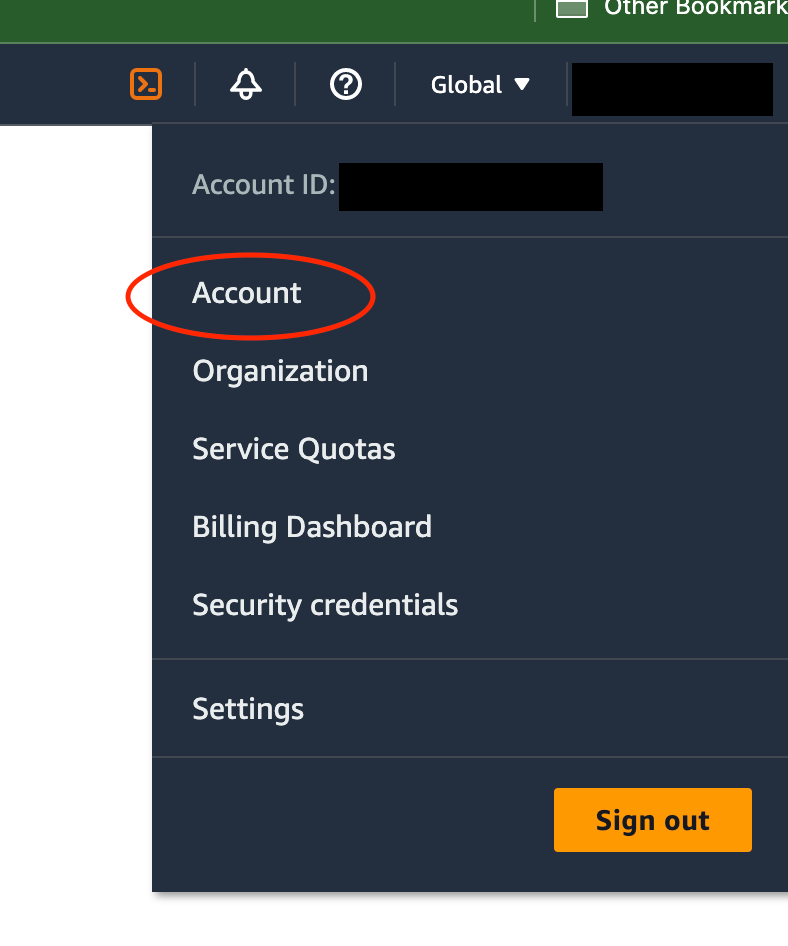
17. Scroll down until you find the “IAM User and Role Acess to Billing Information” section, and click “Edit”
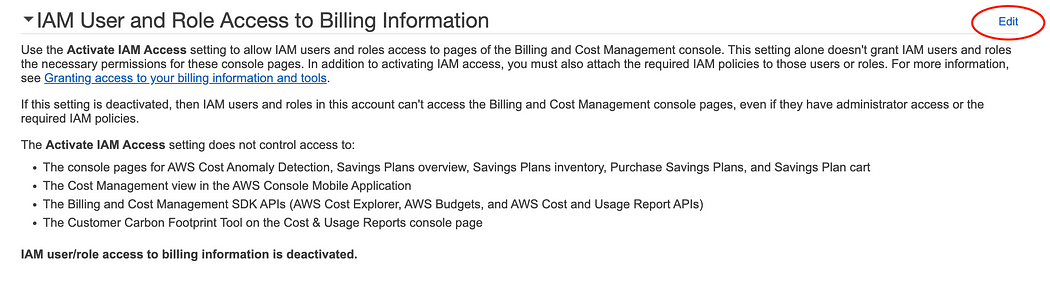
18. A new setting will appear on the bottom, check the box “Activate IAM Access” and click update.
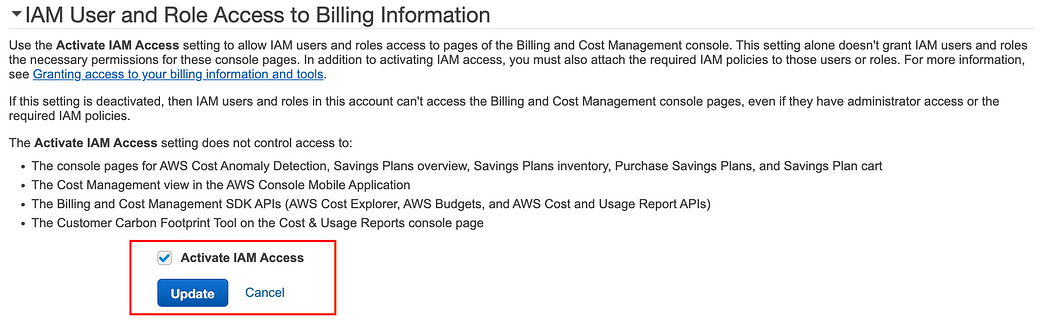
Final step: to proceed with a Well-Architected Framework Review, email both the downloaded files containing the Username, Password, Console sign-in URL, Access key ID, Secret access key to the Solution Architect (or another person) who will work on your account.
AI/ML Transformations in Manufacturing: Boosting Efficiency
AI/ML Transforming Car Manufacturing
These days,when a new car rolls off the line at any major European car manufacturer, chances are, it had a little help from AI. Yep, artificial intelligence is shaking things up in the manufacturing world, making products faster and more accurately than ever before. It's like having a super-efficient robot buddy on the production line!
Ankercloud, a trailblazer in AI technologies, has been at the forefront of this transformation, empowering manufacturing industries to optimize processes, enhance productivity, and minimize downtime. Let's delve into how Ankercloud's AI/ML solutions are revolutionizing the manufacturing sector.
"It's not just about making things; it's about making things smarter."
Streamlining Operations with AI/ML
Ankercloud's expertise lies in harnessing AI algorithms to automate processes and derive actionable insights from vast volumes of data. By leveraging ML models developed specifically for manufacturing environments, Ankercloud enables companies to streamline operations, from supply chain management to production line optimization. Predictive maintenance, a cornerstone of Ankercloud's offerings, utilizes ML algorithms to anticipate equipment failures before they occur, thereby reducing unplanned downtime and maintenance costs.
Enhancing Quality Control
Quality control is non-negotiable in manufacturing, and Ankercloud's AI-powered solutions elevate this critical aspect to new heights. Through image object classification and Optical Character Recognition (OCR) analysis, Ankercloud enables manufacturers to detect defects in real-time, ensuring that only products meeting stringent quality standards reach the market. By automating quality control processes, Ankercloud not only minimizes errors but also accelerates time-to-market for new products.
Optimizing Resource Allocation
Efficient resource allocation is fundamental to maximizing productivity in manufacturing. Ankercloud's AI/ML solutions provide invaluable insights into resource utilization, enabling companies to allocate materials, manpower, and machinery optimally. By analyzing historical data and real-time operational parameters, Ankercloud empowers manufacturers to make data-driven decisions, thereby reducing waste and enhancing overall efficiency.
Predictive Analytics for Demand Forecasting
Anticipating market demand is key to maintaining a competitive edge in manufacturing. Ankercloud's predictive analytics capabilities utilize ML algorithms to forecast demand trends accurately. By analyzing historical sales data, market dynamics, and external factors, Ankercloud enables manufacturers to optimize production schedules, minimize inventory costs, and meet customer demand with precision.
The Business Impact
Ankercloud's AI/ML transformations in manufacturing yield tangible results that directly impact the bottom line. By optimizing processes, enhancing quality control, and enabling predictive analytics, Ankercloud empowers manufacturers to:
Increase Efficiency
Reduced downtime, streamlined operations, and optimized resource allocation lead to enhanced productivity and cost savings.
Improve Quality
Automated quality control processes ensure the delivery of high-quality products, enhancing brand reputation and customer satisfaction.
Optimize Costs
Predictive maintenance and demand forecasting enable manufacturers to minimize maintenance costs, inventory holding costs, and waste, resulting in significant cost savings.
Stay Competitive
By leveraging AI/ML technologies, manufacturers gain a competitive edge through agility, responsiveness, and the ability to adapt to changing market dynamics.
In conclusion, Ankercloud's AI/ML solutions are driving a paradigm shift in the manufacturing sector, empowering companies to embrace digital transformation and thrive in an increasingly competitive landscape. With a focus on efficiency, quality, and innovation, Ankercloud is revolutionizing manufacturing processes and shaping the factories of the future.
How VisionForge impacts on E-Commerce, Retail, Consumer Packaging, and Customer Experience
Product design and development is a booming industry making up the $14,000 million industry globally, and it is only expected to grow. Designers and marketers have an incredibly intricate and important role in the commercialization of products. Bringing a product to market in an appealing, engaging, and eye catching manner is the key to delivering high sales and revenue for an industry. But this task is easier said than done, as designing products, packaging, store arrangements, and online visualizations is a painstaking process taking skilled designers weeks if not months to create. Producing a high quality design that matches the brand, seasonality, and consumer metrics has never been an easy task, but VisionForge from Ankercloud technologies is aiming to make it easier. Let’s examine some of the use cases where VisionForge can streamline the creation process.
1. Visualizing Retail Excellence: VisionForge's Impact on the Customer Experience
In the dynamic landscape of retail, shaping an attracting customer experience is important. The surging demand for immersive shopping encounters has boomed the world's retail market to different heights. Imagine a retail store that sells a wide range of products, including home decor items such as flower vases/pots, clocks, paintings and wallpapers etc. The store aims to create visually appealing product displays to attract and engage customers. However, manually rearranging products in various settings to assess their visual impact on a passing customer can be time-consuming and tedious.
Enter VisionForge. The retailer integrates VisionForge into their merchandising and retail strategy. When introducing a new product, the model is trained to simulate and visualize how the vase or a painting would appear in different contexts within a home setting. The product allows the user to visualize different layouts, lightings, and consumer views, based on what works for them. This includes scenarios like placing the vase on a dining table or in a living room shelf with precise position, color, and mood aptitude.
For example, to promote seasonal or special events, store managers can input specific seasonal themes or events, and VisionForge will generate virtual mock-ups of the store layout tailored to those parameters. For instance, it can visualize how the introduction of holiday-themed decor items or seasonal color palettes will enhance the overall ambiance of the store. This allows retailers to proactively plan and execute visually striking displays that resonate with customers during specific times of the year, encouraging customers to spend more time in stores, driving sales.
This cutting-edge tool kicks off the process by collecting a set of reference images from the user, capturing expansive views of the store or zooming in on specific product displays. Users are then granted creative freedom to experiment with color schemes, merchandise arrangement, lighting, and more, tailoring the visuals to harmonize seamlessly with their distinctive preferences and style. It commences with a specialized text-to-image model, interpreting user prompts and settings to craft sets of images spotlighting key features of the retail space based on the set of reference images that the user has submitted, capturing the style and themes of the images. Following this, customers can refine these images using an image-to-image model equipped with inpainting capabilities, seamlessly integrated with the robust Bedrock API from AWS. This can be used to make major or minor fixes to the images, including changing positioning, adding or removing objects, fixing lighting, or color modifications.
VisionForge, with its adept simulation and visualization capabilities, becomes a catalyst for strategic decision-making. Imagine store managers inputting seasonal themes or special events, and VisionForge responding with virtual mock-ups tailored to those parameters. This isn't just about creating visually appealing displays; it's about driving sales. Retailers, armed with VisionForge, can proactively plan and execute captivating displays during specific times of the year, enticing customers to spend more time in stores and, consequently, increasing sales.
Moreover, the efficiency gains are palpable. From the initial collection of reference images to the refinement process through the image-to-image model, VisionForge streamlines the visual merchandising workflow. It's not just about saving time; it's about optimizing resources and maximizing returns. As retailers experiment with layouts, colors, and lighting within the virtual canvas, they are sculpting an environment that resonates with customers on a deeper level.
The financial implications are clear – VisionForge doesn't merely improve product presentation; it boosts the bottom line. With the power to make major or minor fixes seamlessly, retailers can ensure their displays align with market demands and consumer preferences. This isn't just an investment in aesthetics; it's a strategic move to captivate consumer attention, drive sales, and navigate the ever-evolving retail landscape with a visual prowess that translates into tangible business success. VisionForge isn't just a tool; it's a savvy business ally, transforming the retail narrative and solidifying its place as a strategic imperative in the pursuit of retail excellence. Reference
2. VisionForge: Elevating E-Commerce with Technological Brilliance
In the dynamic realm of e-commerce, where the visual allure of a product can be the make-or-break factor, Ankercloud's VisionForge emerges as a technological masterpiece poised to redefine online retail. This sophisticated image generation platform, fueled by the potent combination of stable diffusion and the Bedrock API, empowers businesses to transcend conventional limitations in product presentation. By seamlessly integrating VisionForge, e-commerce ventures can shatter the barriers imposed by design firms and financial constraints, unleashing the full potential of hyper-realistic packaging ideas with instantaneous materialization.
VisionForge's image-to-image and depth-to-image capabilities enable meticulous adjustments, offering marketers unprecedented control over crucial details such as color schemes, thematic elements, mood, and lighting. The result is a visually striking, attention-grabbing product presentation that seamlessly integrates with the fast-paced nature of the e-commerce landscape.
In the competitive arena of e-commerce, agility is synonymous with success. VisionForge not only accelerates the creative process but also enables rapid iterations with precision. Marketers can experiment with various artistic visions, logos, promotions, and themes within the virtual canvas, fostering an environment where businesses can adapt swiftly to market demands. As a silent ally, VisionForge positions marketers front and center, allowing them to bring their packaging ideas to life efficiently. With a keen focus on business efficiency, VisionForge ensures that e-commerce ventures can navigate the ever-evolving landscape with a visual prowess that captivates consumer attention and drives bottom-line success.
In the fiercely competitive e-commerce arena, the value proposition extends beyond creativity; it's about agility and adaptability. VisionForge not only expedites the creative process but enables marketers to iterate rapidly with precision. This isn't just about experimenting with artistic visions within a virtual canvas; it's about fostering an environment where businesses can swiftly respond to market demands. As a silent yet powerful ally, VisionForge places marketers at the forefront, allowing them to bring packaging ideas to life efficiently and effectively.
The focus on business efficiency is not just a promise; it's a tangible outcome. VisionForge positions e-commerce ventures to navigate the ever-evolving landscape with a visual prowess that not only captivates consumer attention but significantly contributes to driving bottom-line success. Beyond being a technological marvel, VisionForge emerges as a strategic investment, transforming the e-commerce narrative and solidifying its role as a catalyst for sustainable business growth in the digital marketplace.
3. Consumer Packaging
Ankercloud proudly presents VisionForge, a sophisticated image generation platform powered by the cutting edge duo of stable diffusion and the Bedrock API, allowing brands to present their products in the best light. This is not just the future of consumer packaging but a revolutionary idea that shall bridge the gap between the physical and digital world. Personalized packaging not only helps buyers recognize your items, but it also helps them recall their features and the corresponding brand attentively. VisionForge harnesses the power of Generative AI to take in designers’ requests and brand direction to move past the traditional marketing tactics to produce imaginative and engaging product packaging and designs.
With VisionForge, one is no longer constrained by design firms or financial constraints and can create incredible and hyper realistic package ideas that materialize instantaneously, made possible by a custom trained Stable Diffusion model hosted through a Sagemaker endpoint for optimal connectivity and access. VisionForge allows users to upload examples of designs/packaging they are looking to create, and coupled with creative prompting, VisionForge can generate upscaled designs in a matter of seconds. Any errors, misdirects, or faults can then be altered using the image to image and depth to image capabilities of VisionForge allowing the product to make changes in color, theme, mood, and lighting of the product packaging.
In a fast-paced world of consumer goods, packaging is everything. It's the quiet salesman, the initial impression that a product’s packaging makes is vital. However, creating packaging that is visually appealing might involve balancing brand identity, shelf appeal, and practicality. From now on no more endless rounds of physical prototypes and agonizing modifications. Marketers can quickly imagine your package ideas with VisionForge in eye-catching detail and upscaled sharpness. Within the virtual canvas, marketers can impose different artistic visions, colors, logos, promotions, themes, and moods.
Several iterations of a design can be rendered in a matter of seconds with VisionForge with focused precision and customizable levels of guidance and convergence. The product puts the marketer front and center, allowing them to bring their packaging ideas to life.
The efficacy gains extend further, with VisionForge's prowess in swiftly rendering multiple iterations of a design in mere seconds. This unparalleled amalgamation of speed, precision, and customization propels marketers to the vanguard of packaging innovation. VisionForge not only empowers marketers to breathe life into their packaging visions but does so with a level of efficiency that directly impacts the bottom line. In this transformative odyssey, VisionForge stands not just as a testament to technological brilliance but as a discerning business solution that optimizes resources, expedites creative processes, and positions marketers as maestros orchestrating packaging excellence in the fiercely competitive consumer goods landscape.
VisionForge-A effective and efficient solution in the Real Estate and Design Sector
Prospective home buyers have many factors to consider when looking for their dream home; the neighborhood, noise, environment, and most important of all, the interior of the home itself. Creating an appealing image of one’s home has boomed India’s Interior Design market to $31.5 Billion in 2023 [1]. This includes furniture, modeling, and most important of all when it comes time to sell the house: shooting. An interior photoshoot service of a house, the optimal way to attract buyers to view and tour your house, can be expensive and intrusive, requiring hours if not days of on site photography, editing, and further development. Homesellers often have no other avenue to showcase their homes on websites other than availing these services. This is the problem that VisionForge from Ankercloud is aiming to tackle.
VisionForge is a solution that allows homebuyers to develop high quality, photo realistic, and sharp images of their home, given only a small set of reference images, and reference prompts to encourage adherence and consistency. Prospective homebuyers can virtually walk through spaces, exploring every nook and cranny with unparalleled detail. From furniture placement to color schemes, potential buyers can tailor the visuals to align with their unique taste and lifestyle. This level of personalization elevates the home-buying experience. Extending their inquisitive designs to realistic visualizations with the high customization capabilities of VisionForge. This immersive experience transcends conventional property viewing, fostering a deeper connection between potential buyers and their envisioned living spaces.
VisionForge works by taking in a set of reference images from the user first. These can include wide shots covering a large area of rooms, or profile shots, focused on a set of objects closely. The room for experimentation and creativity is given to the user. Then, the user can customize their picture based on different color schemes, furniture layouts, lighting, and more. The solution will use templates of prompts combined with a set of parameters unique to each use case and inference to generate high quality images of customized rooms that fit their vision. This allows the user to paint with broad strokes on their home without ever stepping out of it.
The model works in a two step process, first utilizing a custom trained text to image model that takes in the user’s prompts and settings to generate sets of photorealistic and sharp images that include key features of the rooms/sets in question. Then, the user will be able to edit various aspects of the images, such as colors of certain objects, position, or even the type or style of the objects themselves using an image to image model using inpainting and the Bedrock API from AWS.
Put together, VisionForge is an elegant solution to a prevalent problem, accessible through a single Sagemaker endpoint, robust and flexible enough to handle complex requests and comprehensive product modifications, delivering a stable and highly available product streamlined through the API gateway and Lambda functions. With AWS Bedrock providing a robust foundation, the entire process becomes scalable, reliable, and tailored to the unique demands of various clients and businesses.
VisionForge’s advanced capabilities in image generation bring a new dimension to real estate marketing, offering a personalized and immersive experience for prospective buyers. The tool goes beyond static representations, creating hyper-realistic depictions of interiors that transcend traditional limitations. The ability to visualize spaces in such detail minimizes uncertainties, allowing individuals to make informed decisions confidently. This level of personalization in hand elevates the home-buying experience, making it more engaging and emotionally resonant.
References:
Please Type Other Keywords
The Ankercloud Team loves to listen
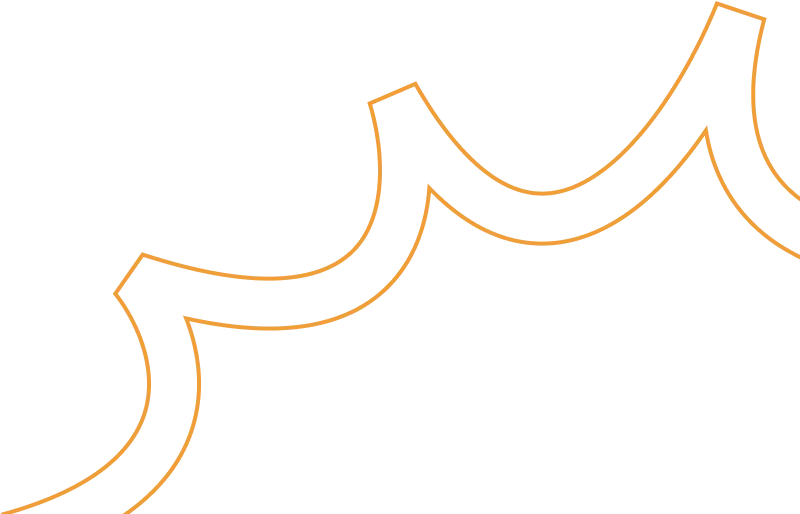
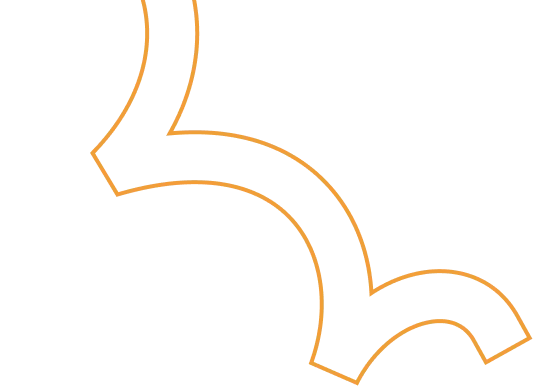