Getting started with an AWS data lake
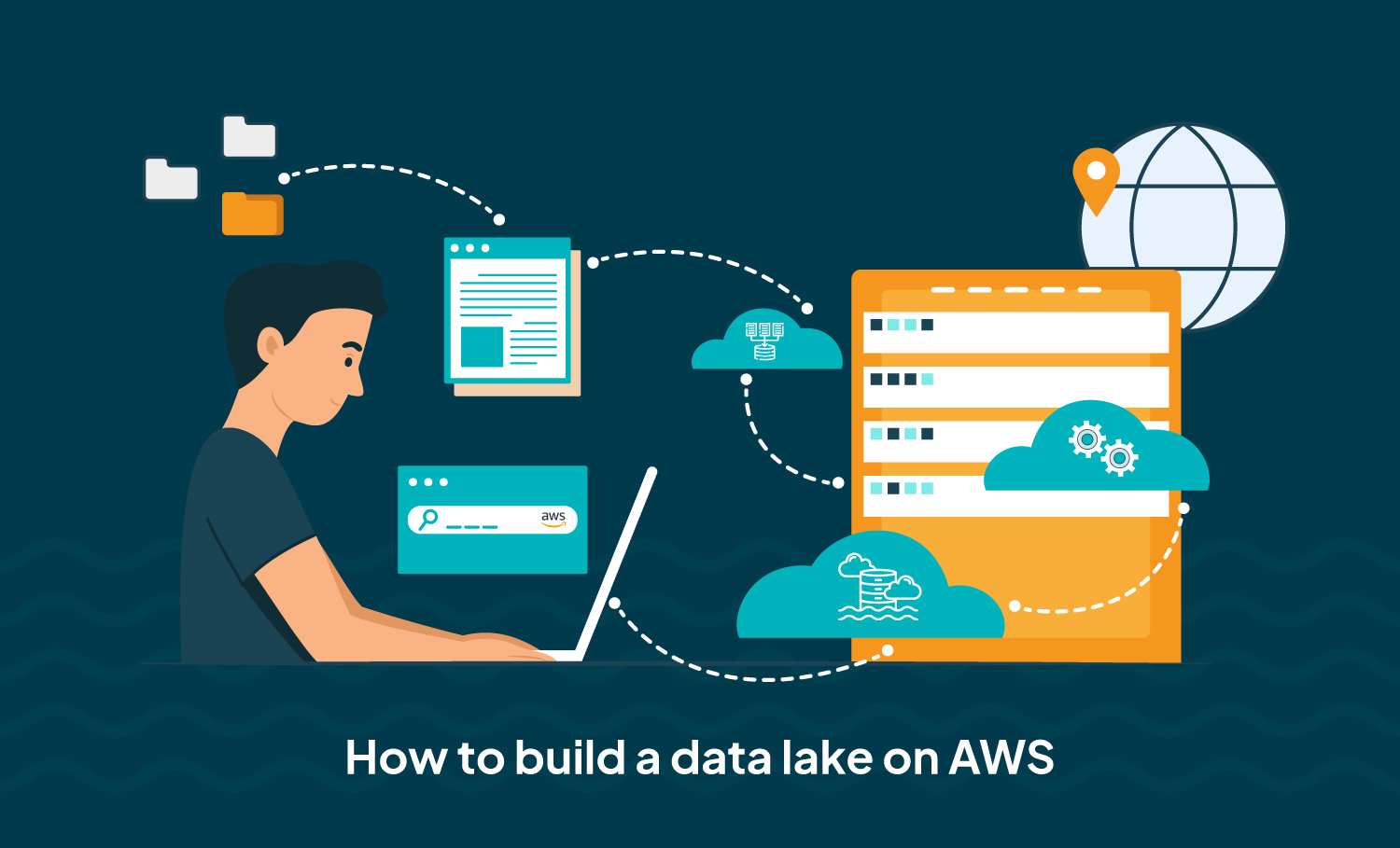
What is Data Lake?
A data lake is a sizable, central repository that enables businesses to store and handle enormous volumes of unstructured, raw data in a variety of formats. Many sources, such as transnational systems, social media, sensors, and more, can contribute data to a data lake.
What is AWS Data Lake?
An organization can store and handle enormous amounts of raw, unstructured data in numerous formats in a data lake, a sizable, centralised repository. A data lake may contain data from many different sources, such as transactional systems, social media, sensors, and more.
Need of Data lake :-
You should construct an AWS Data lake immediately if you are experiencing any of the difficulties listed below.
1. Companies without a single source of data and too many data storage. having trouble obtaining info from several sources.
2. The cost of storing data is out of control and data volume is growing daily.
3. The way that data is organized varies greatly. Businesses, for instance, have data from logs, IoT devices, user audits, and image galleries.
4. Big data analytics using slow data.
This would make it obvious to you whether your company needs Amazon Data Lake or not.
Services Under in AWS DATA LAKE :-
A data lake can be created and managed using a number of capabilities and services provided by Amazon Web Services (AWS). Organizations can store all of their structured and unstructured data in a data lake, which is a central repository that works at any scale. Here are a few characteristics of the Amazon data lake :
Amazon S3 :- The main storage service for constructing data lakes is Amazon S3 (Simple Storage Service). It offers scalable object storage for data of any size and kind.
AWS Glue :- Data transfer between data stores is simple with the help of AWS Glue, a fully-managed extract, transform, and load (ETL) service. Additionally, it has the ability to automatically find and collect metadata related to your data.
AWS Lambda Functions:- In AWS Data Lake, Lambda functions can play a crucial role in automating and enhancing data processing workflows. Data Transformation : Lambda functions can be used to transform data as it is ingested into the Data Lake. Event Processing where Lambda will do automatic processing of data reducing the need for manual intervention.The pay as you go model will help in optimize the costs in Data Lake architecture and Lambda being serverless, can automatically scale up and down to varying workloads.
Amazon Athena :- Data in Amazon S3 may be analyzed using conventional SQL thanks to Amazon Athena, an interactive query service. As there is no infrastructure to set up, it is serverless.
Amazon EMR :- Processing massive volumes of data using distributed frameworks like Hadoop, Spark, and Presto is simple with Amazon EMR (Elastic MapReduce), a fully-managed service.
AWS Lake Formation :- You may quickly and securely create a data lake using the AWS Lake Creation service. Data transformation, data access controls, and categorization are just a few of the functions it offers.
AWS Glue DataBrew :- It’s simple to clean and normalize data for analysis using Amazon Glue DataBrew, a visual tool for data preparation.
Amazon Redshift :- A cloud data warehouse called Amazon Redshift makes it simple to analyze sizable amounts of structured data. It is compatible with additional AWS services like Amazon EMR and AWS Glue.
Amazon Kinesis :- The platform for streaming data on AWS is called Amazon Kinesis. You can use it to gather, process, and analyze streaming real-time data from a variety of sources.
Amazon QuickSight :- For your data lake, it is simple to generate visualisations and dashboards using Amazon QuickSight, a cloud-based business intelligence solution.
These are just a handful of the numerous Amazon data lake functionalities that are offered. They offer an extensive collection of tools for creating, maintaining, and analyzing data lakes at scale when used collectively.
Advantages of AWS Data Lakes :-
You can safely store, examine, and share massive volumes of data at scale with the fully managed service provided by AWS Data Lake. Using AWS Data Lake has a number of benefits, such as:
Scalability :- Petabytes of data may be handled by AWS Data Lake, which scales itself as your data increases.
Cost-effective :- It’s a cost-effective method for handling massive volumes of data because you only pay for the storage and computing resources you really use.
Security :- To protect your data, Amazon Data Lake offers a number of strong security features, including access control, auditing, and encryption both in transit and at rest.
Flexible :- You can choose the appropriate tool for the task by utilizing AWS Data Lake, which supports a number of data formats, including structured, semi-structured, and unstructured data.
Integrations :- You may utilize the ideal tool for the job with Amazon Data Lake since it supports a wide range of data formats, including structured, semi-structured, and unstructured data.
Analytics :- Many analytics tools, like Amazon Athena, Amazon EMR, and Amazon Redshift, are available through AWS Data Lake, making it simple to query and analyze your data.
Collaboration :- When working with coworkers and business partners, Amazon Data Lake makes it simple to securely share data with other users and applications.
AWS Data Lake Architecture :-
Organizations can store, manage, and analyze vast amounts of data from several sources using the scalable and secure AWS Data Lake data repository. The architecture of AWS Data Lake typically consists of the following components:
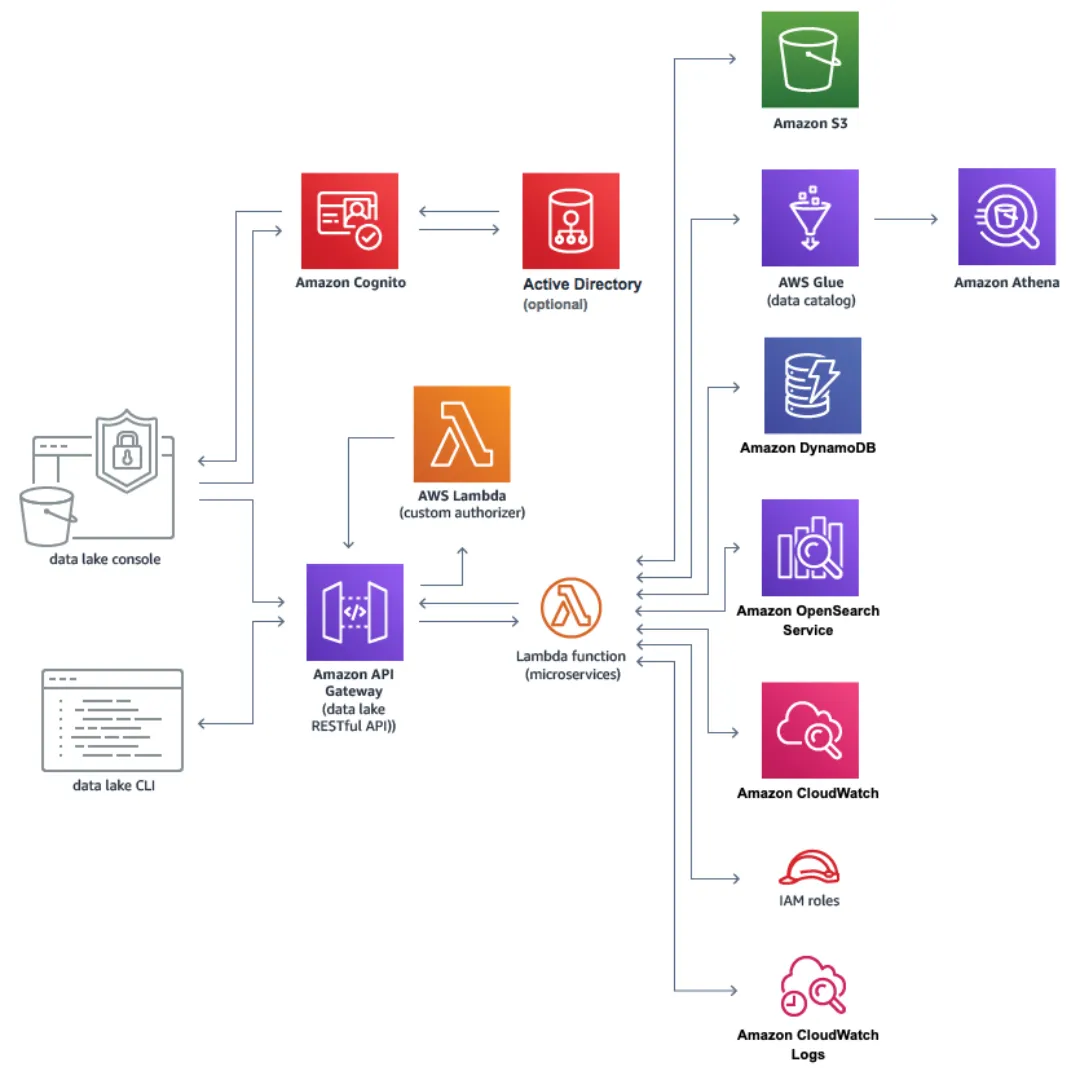
Data Sources :-
Data from several sources, including databases, applications, IoT devices, and social media platforms, can be ingested by AWS Data Lake. These data sources could be local or online.
Data Ingestion :-
AWS offers a number of services, including Amazon Kinesis, AWS Glue, and AWS Data Pipeline, for importing data into the Data Lake.
Data Storage :-
Amazon S3, Amazon EBS, and Amazon Glacier are just a few of the storage solutions that AWS Data Lake provides. With its limitless scalability, superior durability, and affordable pricing, Amazon S3 is the most widely used storage option.
Data Catalog :-
Users may find, comprehend, and manage the data that is stored in the Data Lake using the data catalogue that is offered by AWS Glue. Column names, table definitions, and other metadata are included in the data catalogue.
Data Processing :-
For processing data kept in the Data Lake, AWS offers a number of services like Amazon EMR, AWS Glue, and Amazon Athena. These services can be utilized for activities including data analysis, data cleansing, and data transformation.
Data Visualization :-
AWS offers a number of services for displaying data from the Data Lake, including Amazon QuickSight, which enables customers to build interactive dashboards and reports.
Security and Governance :-
For the protection of the privacy, accuracy, and accessibility of the data kept in the Data Lake, AWS offers a number of security and governance capabilities. Encryption, access management, and audit recording are some of these characteristics.
All things considered, the design of AWS Data Lake offers a highly scalable, safe, and economical option for storing and processing huge volumes of data.
limitations of AWS Data Lakes :-
AWS Data Lake has a lot of benefits, but there are a few potential drawbacks to take into account as well:
Complexity :- It can be difficult to set up and administer an Amazon Data Lake, especially if you are unfamiliar with the AWS ecosystem.
Cost :- While AWS Data Lake can be inexpensive, if you plan to store a lot of data or make a lot of queries, this cost-effectiveness may not last.
Expertise :- You might need to have knowledge of data engineering, data architecture, and data analytics to make the most of AWS Data Lake.
Integration :- While many Amazon services are compatible with AWS Data Lake, not all third-party programs or data sources may be compatible with it.
Latency :- There can be some latency while accessing and searching your data, depending on how you configure your AWS Data Lake.
Maintenance :- Amazon Data Lake needs regular maintenance, just like any other IT system, to guarantee optimum performance and security. It may take a lot of time and resources to do this.
When deciding whether to use AWS Data Lake for your particular use case, it is crucial to balance these potential drawbacks with the advantages of doing so.
Conclusion :-
In general, AWS data lake offers a wide range of advantages, such as streamlined data administration, enhanced data quality and accessibility, accelerated time to insights, and cost savings. But setting up and maintaining an AWS data lake requires knowledge of data management and AWS services, so it’s crucial to carefully plan and design the architecture to make sure it satisfies the organization’s unique requirements.