AI & ML Solution for a Facade Building Company
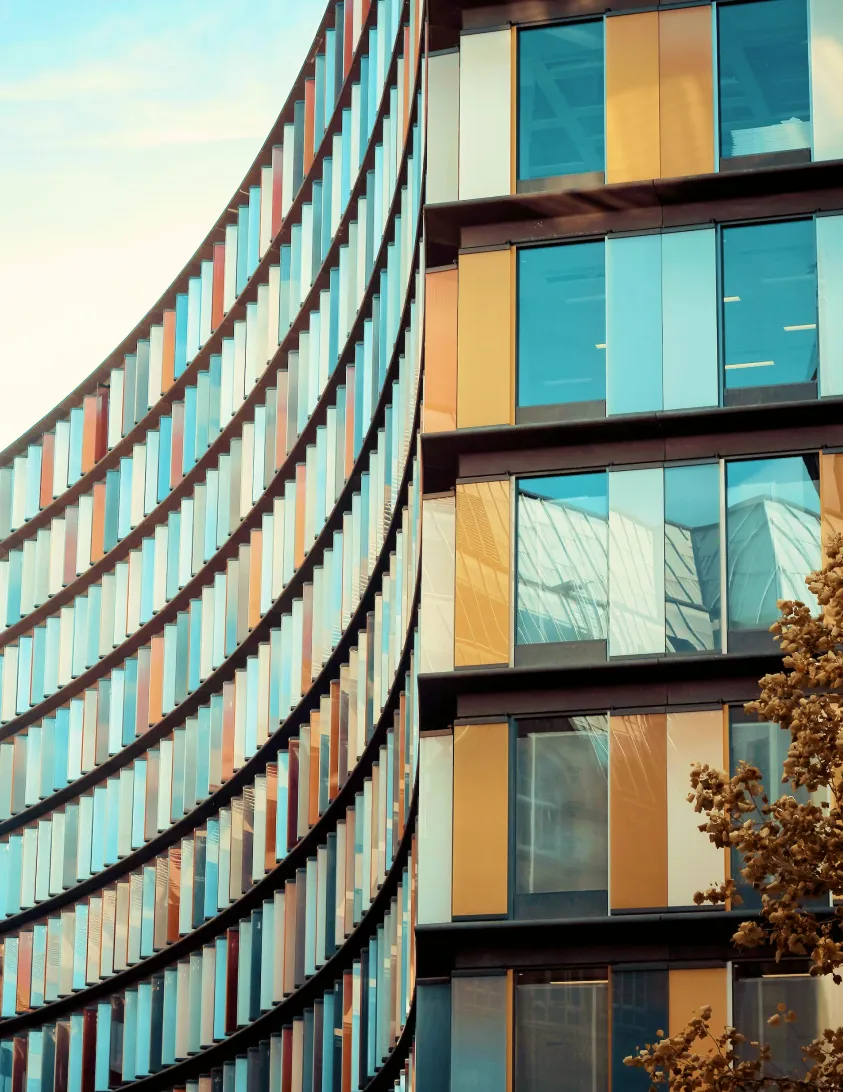
Key Challenges
Federmeister, a facade building company, faced a major challenge with their inefficient quote generation process. Customers uploaded images for quotes, which were then converted into different formats using a third-party tool for cost calculation. This laborious process, averaging 8 hours, often failed to meet deadlines during high-demand periods, impacting customer satisfaction and operational efficiency.
Key Results
Federmeister addressed their slow quote generation process by deploying an AI and ML solution that automatically detects, classifies, and measures facade elements from uploaded images, cutting down the processing time from two weeks to just 8 hours. The system, trained on extensive datasets, achieves about 80% accuracy in identifying facade components. This significant upgrade not only reduced manual labor but also enhanced the company's ability to handle workload fluctuations, greatly improving operational efficiency and responsiveness.
Overview
AI & ML Solution for a Facade Building Company
Federmeister specializes in the renovation of building facades. With a set of experienced workforce, they are called a specialist company in the market for quality in all trades: from planning to execution on the construction site. Federmeister has developed a digital platform for customers to quickly upload their requirements and get a customized quote for the desired services. They provide personal advice to customers with facade experts. Currently, they operate in four regions Rhine-Main metropolitan, Central Germany, Rhine-Neckar metropolitan and Stuttgart) In the near future, the rollout to the whole of Germany is planned.
Challenges
At present, customers upload images on the platform to request a quote on the desired services. Once Federmeister’s backend team receives the information, they evaluate the requested services by using a third-party tool to re-create the image in different formats and calculate the cost of the services. This process takes an average of 8 hours in conversion which will help to plan and calculate the total cost and send the quote. When the inquiries are high on certain days, the actual deadline for sharing quotes with the customer is hard to achieve.
Solution
- Built an application to compare the area with the predefined fixed values (with reference sheet) and calculate a quote. It will enable customers to upload correct images of their facades to the given web application.
- Ability to detect objects and classify correctly and provide an accurate bounding box for the detected object.
- The developed machine learning engine will detect and classify the door, windows, and facade. A customized algorithm is developed specifically to identify the doors, windows, and facades and trained on the engine.
- The ML engine detects the image and measures the area of the objects (door, windows, and facade) with the predefined fixed values (with a given reference sheet) and calculates a quote based on the measurements.
- The required measurement method & quote formulas will be prefixed in the system backend. The output will be given in the desired format requested by the client.
Business Outcome
- Each component of the facade like windows, doors, and wall are trained with a large number of datasets (shared from Federmeister) to give high accuracy.
- Time taken for quote generation reduced drastically from 2 weeks to 8 hours. This reduced the manual effort being put in and increased operational efficiency.
- Gives the capacity to change in size or scale without worrying about the workload.
- We expect the solution to achieve 80% accuracy in identifying the facade (door, window, wall). We will measure the objects with pre-defined values, and give the cost calculation as output in the desired format.