Resources
The latest industry news, interviews, technologies and resources.
Navigating the Cloud Securely: A Deep Dive into Cloud Security Measures
Cloud computing has revolutionized the way businesses operate, providing scalability, flexibility, and cost-efficiency. However, this transformation has also exposed organizations to a new wave of security challenges. The shared responsibility model adopted by most cloud providers demands that businesses take active measures to secure their data and applications.
Cloud Security Challenge
While cloud technology offers remarkable advantages, its distributed nature introduces a unique set of security challenges:
Data Breaches: Cloud breaches can result in significant data exposure. Whether due to misconfigured settings or insider threats, unauthorized access to sensitive data remains a top concern.
Insecure APIs: Application Programming Interfaces (APIs) facilitate interaction between various cloud components. Inadequately secured APIs can serve as entry points for attackers.
Shared Responsibility: Public cloud providers operate on a shared responsibility model, where they secure the infrastructure while customers are responsible for securing their applications and data.
Compliance and Governance: Organizations must ensure that their cloud infrastructure adheres to industry regulations and internal security policies.
Our Cloud Security Offerings
We take a holistic approach to cloud security, combining cutting-edge technology and industry best practices to create a multi-layered defense strategy. Our services include:
Risk Assessment and Best Practices Compliance: Our experts conduct thorough risk assessments to identify vulnerabilities within your cloud infrastructure. We ensure compliance with best practices, industry standards, and regulations, providing you with a roadmap to enhance your security posture.
Data Encryption: Protect your sensitive data from unauthorized access with state-of-the-art encryption solutions. Our encryption techniques ensure that even in the event of a breach, your data remains unintelligible to malicious actors.
IAM Users Management: We check policies and permissions of your users to give you a full overview who has access to which resources, what changes they are allowed to implement and which are the risks associated. With ongoing monitoring and management, you gain complete clarity on users’ actions.
Multi-Factor Authentication (MFA): Strengthen your access controls with multi-factor authentication. By adding an extra layer of verification, MFA prevents unauthorized users from gaining access to your cloud resources.
Intrusion Detection and Prevention: Our advanced intrusion detection systems actively monitor your cloud environment, promptly identifying and mitigating any suspicious activities to prevent potential breaches.
Security Incident Response: In the unfortunate event of a security breach, Ankercloud is by your side. We have a well-defined incident response protocol to minimize damage, recover data, and prevent future incidents.
Continuous Monitoring: Cloud security is an ongoing process. We help you leverage the available cloud native tools for adequate monitoring, long with provide continuous management to keep your cloud environment secure against emerging threats.
Secure Your Cloud with us
Don't compromise on your cloud security. Choose Ankercloud Cloud Security Service and fortify your digital infrastructure against emerging threats. Contact us today to learn how our tailored solutions can elevate your cloud security strategy, enabling you to embrace the cloud's potential while keeping your data secure. Partner with us for a secure digital journey into the future.
Generative Artificial Intelligence (GenAI)
Innovative Algorithms: Generative AI Reshaping Industries
One of the most fascinating areas within AI is Generative Artificial Intelligence (GenAI), a technology that enables computers to create content that ranges from images and music to text and even code. Public cloud providers, such as Amazon Web Services (AWS), Google Cloud Platform or Microsoft, have made significant strides in this field with its Generative Artificial Intelligence (GenAI) offerings.
Generative Artificial Intelligence involves the use of machine learning algorithms to create original content, imitating human-like creativity. These algorithms are trained on vast datasets and can produce everything from images and music to text and video. The cornerstone of GenAI is its ability to learn patterns and relationships from existing data and generate new content that adheres to those patterns.
Ankercloud stands at the forefront of leveraging GenAI offerings to unlock unparalleled creative potential. By combining cutting-edge technology with Ankercloud’s expertise, businesses can harness the power of Generative AI to transform their operations and captivate their target audience.
The Power of AWS GenAI Services
With Ankercloud’s GenAI services, the possibilities are as diverse as they are exciting:
Custom GenAI Models
Our team of experienced AI professionals collaborates closely with your business to create bespoke Generative AI models. These models are finely tuned to cater to your unique content creation needs, whether it's generating images, videos, text, or even music.
AI Workload Expertise
Ankercloud is well-versed in premier machine learning service from AWS and GCP. We utilize workloads like Amazon SageMaker to develop, train, and deploy GenAI models, ensuring your business stays at the forefront of AI-driven innovation.
Diverse Industry Applications
Our GenAI services transcend industry barriers. Whether you're in e-commerce, design, entertainment, healthcare, or gaming, Ankercloud has the expertise to tailor GenAI solutions that align with your business objectives.
Ankercloud's GenAI Experience: Tangible Insights from Successful Projects
Ankercloud has successfully implemented several projects, in collaboration with AWS and GCP, to provide tangible solutions for various customers. Here are a few detailed examples:
1. Customer Support Chatbot
- Verticals: EdTech, Commercial & Governance
- Challenge: A global language solutions startup faced delays and management issues due to manually handling user queries.
- Solution: Ankercloud's solution involved developing an AI-driven chatbot using Amazon Lex, Amazon Kendra, and Amazon Connect. This bot autonomously provides answers, directs users to the right department, and improves over time.
- Outcome: Reduced expenses, quicker support, enhanced customer satisfaction, and improved agent capabilities.
2. Public Sector Chatbot
- Challenge: customer in the German Public Sector needed an AI-driven chatbot for its website, offering quick responses, natural conversations, and global contact information.
Solution: Ankercloud built a conversational chatbot using AWS services like Amazon Lex, Lambda function, and API Gateway.
-Outcome: The chatbot effectively captures user intent, retrieves data from a PostgreSQL database, and provides tailored responses.
3. Automatic Report Generation
- Verticals: Insuretech, Healthcare
- Challenge: A Health Insurance Startup dealt with manual and time-consuming processes in handling insurance claims.
-Solution: Ankercloud automated this process through Optical Character Recognition (OCR), validation models, and report generation.
- Outcome: Doctors can quickly access key decision-making data points, treat more patients, and efficiently handle incoming documents.
Forecasted Projects
Ankercloud is actively engaged in several ongoing projects:
- Generating synthetic data for ML model training.
- Developing a recommendation engine for SaaS applications.
- Crafting an NLP chatbot integrated with popular messaging services.
- Creating custom content for educational applications.
These tangible case studies showcase Our expertise in utilizing GenAI to address challenges across various industries, delivering efficiency, cost savings, and enhanced user experiences. Through innovative solutions and the power of collaboration, We are shaping the future of AI-driven transformation.
Conclusion
Ankercloud's utilization of AWS Generative Artificial Intelligence (GenAI) services represents a paradigm shift in the way businesses innovate and create. By embracing this technology, organizations can unlock new levels of efficiency, creativity, and competitiveness. Partner with Ankercloud today to embark on a journey of AI-powered transformation and secure a brighter, more innovative future for your business.
Introducing Ankercloud's BI in a BOX Solution - Unleash the Power of Data!
Are you tired of sifting through endless data, struggling to make sense of it all? Say goodbye to the complexities of data analysis and welcome the future of business intelligence with open arms. Ankercloud presents BI in a BOX - the all-in-one solution designed to empower your business decisions and supercharge your growth.
What is Ankercloud's BI in a BOX Solution?
Ankercloud's BI in a BOX Solution is a comprehensive Business Intelligence (BI) package designed to streamline your data analysis processes. It provides a complete end-to-end solution for collecting, processing, visualizing, and extracting valuable insights from your data,. It's your one-stop-shop for data driven decision, all within a user-friendly and customizable interface.
Why Choose BI in a BOX Solution?
🔍 Unlock Insights, Drive Success:
In today's fast-paced business landscape, making informed decisions is the key to staying ahead. With Ankercloud's BI in a BOX Solution, you gain a bird's-eye view of your data, allowing you to uncover valuable insights that drive growth and innovation. No more guesswork – just data-driven excellence!
📊 All-in-One Analytics:
BI in a BOX is your one-stop-shop for comprehensive analytics. From sales trends and customer behavior to operational efficiency and financial performance, our solution empowers you with the tools to explore, visualize, and understand your data like never before. Make smarter choices, faster.
🌐 Seamless Integration:
Say goodbye to data silos! Our BI in a BOX Solution seamlessly integrates with your existing systems, ensuring that your data is always up-to-date and accurate. Whether it's CRM, ERP, or other software, we bring your data together for a holistic view of your business operations.
📈 Customizable to Your Needs:
Every business is unique, and so are your analytical needs. Ankercloud's solution is highly customizable, allowing you to tailor your analytics dashboards to match your specific industry, goals, and KPIs. No cookie-cutter solutions here – just insights that matter.
🔒 Security You Can Trust:
At Ankercloud, security is paramount. BI in a BOX employs top-tier encryption and data protection measures, ensuring that your sensitive business information is kept safe and confidential. Focus on your data exploration; we'll take care of the security.
🎯 Competitive Edge:
Stay ahead of your competition by leveraging the power of data. With BI in a BOX, you gain the insights needed to identify emerging trends, capitalize on new opportunities, and fine-tune your strategies for maximum impact. It's not just about being in the game – it's about leading it.
📢 Elevate Your Business with Ankercloud
Don't let valuable insights remain hidden in your data. With Ankercloud's BI in a BOX Solution, you'll elevate your business game and uncover opportunities you never knew existed. Embrace the future of business intelligence – get started with BI in a BOX now!
Streamline Your Development with Ankercloud's DevOps and MLOps Pipeline Solutions
In today's fast-paced technological landscape, staying ahead of the competition requires seamless integration, rapid deployment, and efficient management of development and machine learning operations. Ankercloud presents DevOps and MLOps Pipeline Solutions – a game-changing approach to streamlining your workflow, reducing bottlenecks, and achieving peak operational efficiency. In this article, we explore how Ankercloud's innovative pipeline solutions can revolutionize your development workflow.
Benefits of Ankerclouds DevOps and MLOps Pipeline Solutions
Enhanced Efficiency and Speed:
Ankercloud’s DevOps and MLOps Pipeline solutions are designed to accelerate your development lifecycle. With streamlined automation and continuous integration/continuous deployment (CI/CD) pipelines, your team can reduce manual interventions, leading to faster code deployment and quicker time-to-market for your applications and models.
Seamless Collaboration:
Collaboration is at the heart of successful development. Our Solution provides a collaborative platform where developers, testers, and data scientists can work together seamlessly. Shared pipelines, version control, and integration with popular project management tools foster a culture of teamwork, resulting in efficient problem-solving and improved software quality.
Reliable Testing and Quality Assurance:
We ensure that every code change and model update goes through rigorous testing and quality assurance processes. Automated testing environments, code reviews, and integration with testing frameworks guarantee that your software remains stable, secure, and bug-free.
Scalability and Flexibility:
As your projects grow, so do your infrastructure needs. Our solutions scale with your requirements, allowing you to handle larger workloads without compromising on performance. Whether you're managing a small application or a complex machine learning pipeline, Ankercloud solutions adapt to your needs.
Advanced Monitoring and Insights:
Gain deep insights into your development and deployment processes with comprehensive monitoring and analytics tools. We provide real-time performance metrics, logs, and reports, enabling you to make data-driven decisions to optimize your pipelines for maximum efficiency.
Secure and Compliant Practices:
Security and compliance are paramount in today's data-driven landscape. Ankercloud incorporates robust security features into its solutions, including access controls, encryption, and compliance with industry standards. Focus on your development while we take care of safeguarding your data and applications.
Simplified MLOps:
For teams working on machine learning projects, Ankerclouds' MLOps Pipeline solutions offer specialized features. From data preprocessing and model training to deployment and monitoring, We streamlines the end-to-end machine learning workflow, ensuring reproducibility and efficiency in your AI projects.
Conclusion:
Ankercloud's DevOps and MLOps Pipeline solutions offer a transformative approach to modern development practices. By automating key processes, fostering collaboration, and optimizing deployment, our pipelines empower your teams to focus on innovation and deliver high-quality software and machine learning solutions faster than ever before. Embrace the future of development with Ankercloud's cutting-edge pipeline solutions. Contact us today to learn how we can elevate your development workflow.
Migration Acceleration Program (MAP)
The AWS Migration Acceleration Program (MAP) is a structured framework designed to facilitate seamless migration of workloads to the AWS Cloud. The program is divided into three essential phases:
1. Migration Readiness Assessment:
In this initial phase, organizations evaluate their existing IT landscape to determine which workloads are suitable candidates for migration. This involves analyzing dependencies, performance requirements, and potential challenges. By assessing their readiness, organizations gain a clear understanding of the scope and complexity of the migration project.
2. Migration Planning and Mobilize Your Resources:
Once the assessment is complete, organizations move on to the planning phase. This involves creating a comprehensive migration strategy that outlines the approach for each workload. Key considerations include selecting the appropriate migration methods (rehost, re-platform, refactor), estimating costs, and defining a timeline. Mobilizing resources involves assembling the necessary teams, tools, and technologies to execute the migration plan effectively.
3. Migrate and Modernize Your Workloads:
The final phase is the execution of the migration plan. Workloads are migrated to the AWS Cloud based on the selected strategies. This phase also provides an opportunity to modernize and optimize workloads, taking advantage of AWS services and best practices to enhance performance, scalability, and cost efficiency. The focus is on ensuring that the migrated workloads fully leverage the benefits of the AWS Cloud environment.
Throughout these phases, the AWS Migration Acceleration Program offers guidance, tools, and resources to streamline the migration process and enable organizations to achieve their cloud adoption goals efficiently and effectively. As technology and offerings continue to evolve, the program remains adaptable to deliver successful outcomes for businesses embracing the AWS Cloud.
DevOps Trends: CI/CD Automation
CI/CD (Continuous Integration/Continuous Delivery) automation is a crucial aspect of DevOps practices and has been gaining significant attention in recent years. By automating the CI/CD pipeline, organizations can accelerate software delivery, improve code quality, and enhance collaboration between development and operations teams. Here are some notable trends in CI/CD automation:
- Shift-Left Testing: Shift-left testing emphasizes early and continuous testing throughout the software development lifecycle, starting from the earliest stages of development. By integrating testing into the CI/CD pipeline and automating the testing process, organizations can identify and address issues more quickly, reducing the risk of defects reaching production.
- Infrastructure as Code (IaC): Infrastructure as Code is a practice that enables the automation and management of infrastructure resources using code. With IaC, infrastructure configurations can be version-controlled, tested, and deployed alongside application code. CI/CD automation tools integrate with IaC frameworks such as Terraform or AWS CloudFormation to provision and manage infrastructure resources in a consistent and repeatable manner.
- Cloud-Native CI/CD: As organizations increasingly adopt cloud computing and containerization technologies, CI/CD pipelines are evolving to support cloud-native applications. Tools like Kubernetes and Docker are commonly used to build, deploy, and orchestrate containerized applications. CI/CD automation platforms are adapting to support the unique requirements of cloud-native environments, enabling seamless integration with container registries, orchestrators, and serverless platforms.
- Machine Learning/AI in CI/CD: Machine learning and AI techniques are being applied to CI/CD automation to optimize various aspects of the software delivery process. For example, AI-based algorithms can analyze code quality, identify patterns, and provide recommendations for improvements. Machine learning models can also be used to predict and detect anomalies in CI/CD pipelines, enabling proactive identification of potential issues.
- Low-Code/No-Code CI/CD: The rise of low-code/no-code development platforms has extended to CI/CD automation as well. These platforms provide visual interfaces and pre-built integrations that simplify the setup and configuration of CI/CD pipelines, reducing the need for extensive coding or scripting. Low-code/no-code CI/CD tools empower non-technical stakeholders to participate in the automation process and accelerate the delivery of applications.
Benefit of CI/CD: -
· Increased delivery speed & cooperation
· Instantaneous feedback
· Simple to maintain & Reliable
Components of a CI/CD Pipeline: -
a. Jenkins Pipeline: Jenkins Pipeline is a powerful and flexible way to define your continuous integration and continuous delivery (CI/CD) workflows in Jenkins. It allows you to define your build, test, and deployment stages as code, providing a consistent and repeatable process for your software development lifecycle. Jenkins Pipeline supports two syntaxes: Declarative Pipeline and Scripted Pipeline.
i. Declarative Pipeline: Declarative Pipeline provides a more structured and opinionated syntax for defining pipelines. It is recommended for most use cases as it offers simplicity and readability. Here’s an example of a simple Declarative Pipeline:
pipeline { agent any
stages { stage(‘Build’) { steps { // Perform the build steps here } }
stage(‘Test’) { steps { // Run your tests here } }
stage(‘Deploy’) { steps { // Deploy your application here }}}}
In this example, the pipeline has three stages: “Build,” “Test,” and “Deploy.” Each stage contains the necessary steps to be executed.
ii. Scripted Pipeline: Scripted Pipeline provides a more flexible and programmatic way to define your pipelines using Groovy scripting. It allows you to have greater control over the execution flow and provides more advanced features. Here’s an example of a simple Scripted Pipeline:
node {
stage(‘Build’) { // Perform the build steps here}
stage(‘Test’) { // Run your tests here }
stage(‘Deploy’) { // Deploy your application here }}
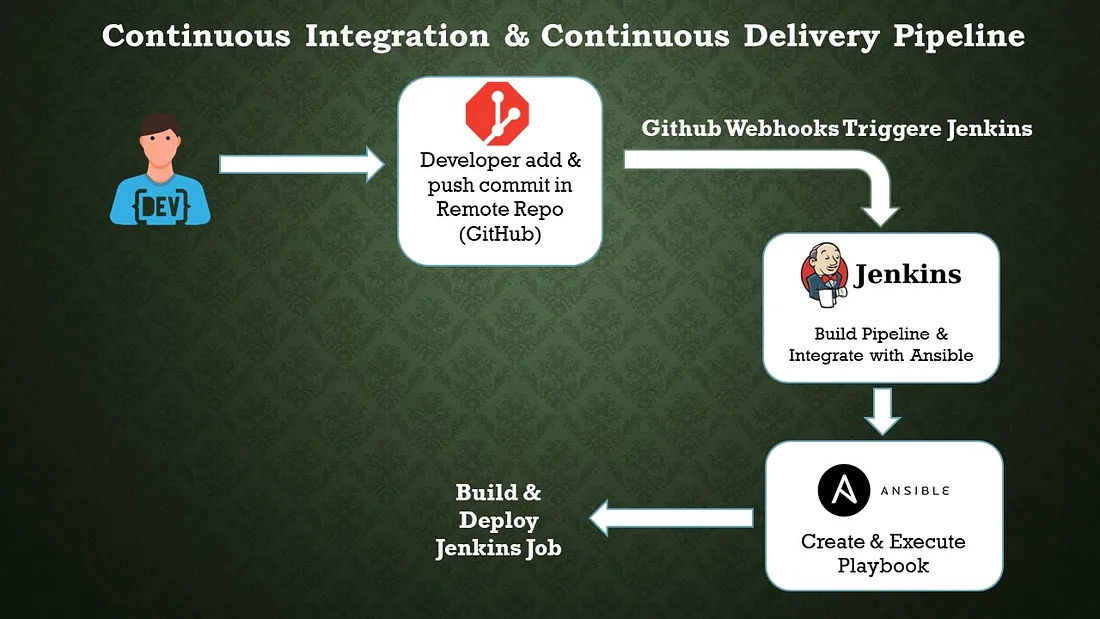
b. Configuration Management Tool: Ansible
Ansible is an open-source configuration management tool that automates the deployment, orchestration, and management of software applications and infrastructure. It is designed to be simple, agentless, and easy to use, making it popular among system administrators and DevOps teams. Here are some key features and concepts related to Ansible:
i. Agentless: Ansible does not require any agents or additional software to be installed on the target systems. It uses SSH (Secure Shell) and Python to communicate with remote hosts, which simplifies the setup process and reduces the overhead on managed systems.
ii. Declarative Language: Ansible uses a YAML-based language called Ansible Playbooks to define configurations and automate tasks. Playbooks are human-readable and describe the desired state of the systems. This declarative approach allows for idempotent execution, where running the same playbook multiple times produces consistent results.
iii. Inventory: Ansible uses an inventory file to define the hosts or systems it manages. The inventory can be a static file or generated dynamically from various sources, such as cloud providers or external scripts. It allows you to organize hosts into groups and apply different configurations to specific groups or individual hosts.
iv. Modules: Ansible comes with a wide range of built-in modules that perform specific tasks, such as managing packages, configuring services, manipulating files, or executing commands. Modules are written in Python and can be extended or customized to meet specific requirements.
v. Playbooks: Playbooks are the heart of Ansible. They are YAML files that define a set of tasks to be executed on remote hosts. Playbooks specify the desired state of the systems, and Ansible takes care of bringing them into that state. Playbooks can include variables, conditionals, loops, and handlers to perform complex configuration management.
vi. Idempotency: Ansible’s idempotent nature ensures that running the same playbook multiple times does not cause unintended changes. If a system is already in the desired state, Ansible skips the corresponding tasks, resulting in a consistent and reliable configuration management process.
vii. Ad-hoc Commands: Ansible allows you to execute ad-hoc commands directly on remote hosts without the need for writing a playbook. This feature is useful for quick troubleshooting, one-time tasks, or running simple commands across multiple systems simultaneously.
viii. Ansible Galaxy: Ansible Galaxy is a hub for sharing and discovering Ansible roles. Roles provide a way to organize and reuse playbook logic, making it easier to manage complex configurations. Ansible Galaxy allows you to find pre-built roles contributed by the community, helping you accelerate your automation efforts.
Conclusion:
Code quality is increased and changes are provided rapidly with CI/CD automation. The automation technique has a very good quality, bug-free, and quicker fault isolation impact. We completed every step of the automation process, including create, build, test, and deliver. Process automation is necessary for software development.
DORA Metrics for DevOps Performance Tracking
INTRODUCTION TO DORA METRICS:
This blog is to explain the DevOps Research and Assessment capabilities to understand delivery and operational performance for better organizational performance.
DORA — DORA (DevOps Research and Assessment) metrics help us to measure the DevOps performance if there are low or elite performers. The four metrics used are deployment frequency (DF), lead time for changes (LT), mean time to recovery (MTTR), and change failure rate (CFR).
The four essentials of DORA metrics:
- Deployment frequency
- Lead time for changes
- Mean time to recovery
- Change failure rate
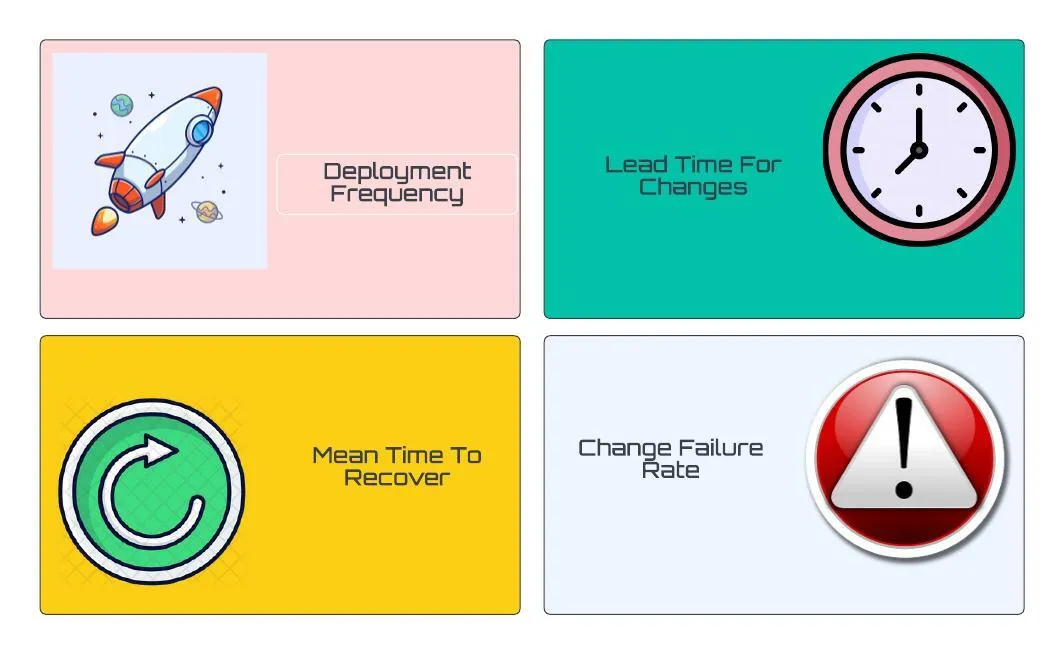
Deployment Frequency :
Deploy frequency measures how often you deploy changes to a given target environment. Along with Change lead time, Deploy frequency is a measure of speed.
Deployment Frequency also provides us with Batch Size Breakdowns,
Allowing you to filter the code changes based on Small batch, Medium, Large, and Gigantic batch sizes.
- Small — usually 1 pull request, 1–10 commits, and a few hundred lines of code changed
- Medium — usually 1–2 pull requests, 10–30 commits, and many hundreds of lines of code changed
- Large — usually 2–4 pull requests, 20–40 commits, and many hundreds of lines of code changed
- Gigantic — usually 4 or more pull requests or 30 or more commits or many thousands of lines of code changed.
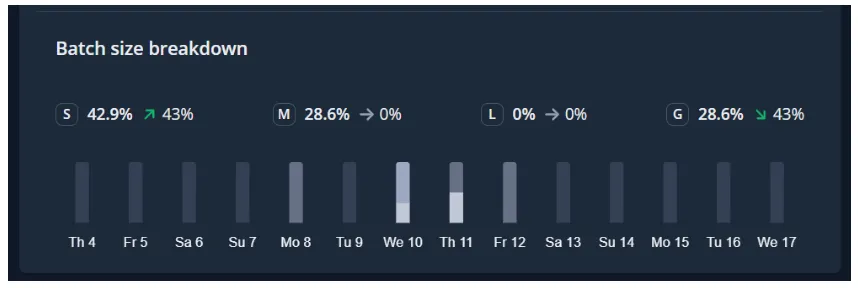
Lead Time for Change:
Change lead time measures the time it takes for a change to go from its initial start of coding to being deployed in its target environment. Like Deploy frequency, Change lead time is a measure of speed (whereas Change failure rate and MTTR are measures of quality or stability).
In addition to the Lead Time for Change, Sleuth provided us with a detailed breakdown of how much time your teams, on average, are spending.
- Coding — the time spent from the first commit (or the time spent from the first transition of an issue to an “in-progress state) to when a pull request is opened
- Review lag time — the time spent between a pull request being opened and the first review
- Review time — the time spent from the first review to the pull request being merged
- Deploying — the time spent from pull request merge to deployment
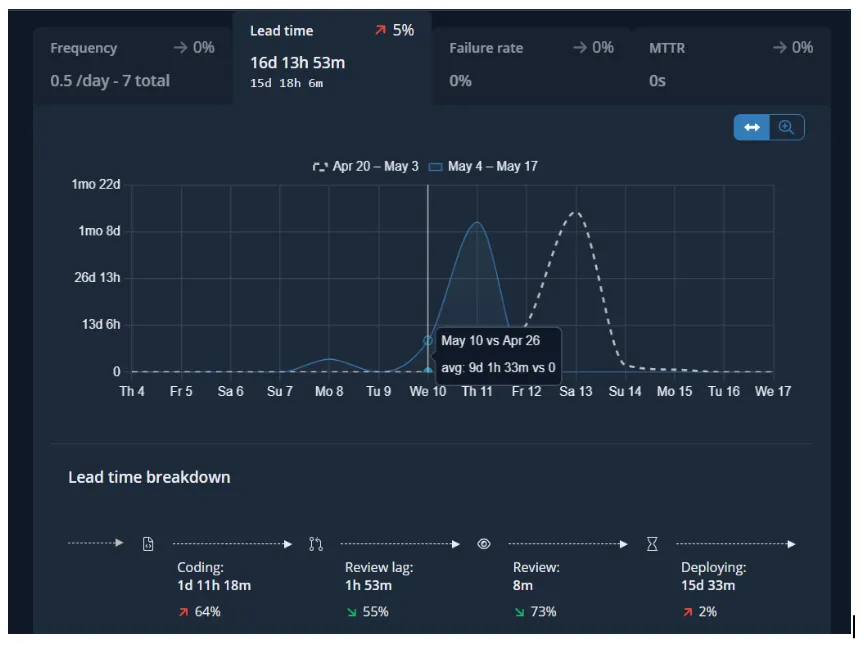
Mean Time to Recovery:
Change failure rate measures the percentage of deployed changes that cause their target environments to end up in a state of failure. Along with MTTR, Change failure rate is a measure of the quality, or stability of your software delivery capability.

Change Failure Rate
Measures the quality and stability while deployment frequency and Lead Time for Changes don’t indicate the quality of the software but just the velocity of the delivery.
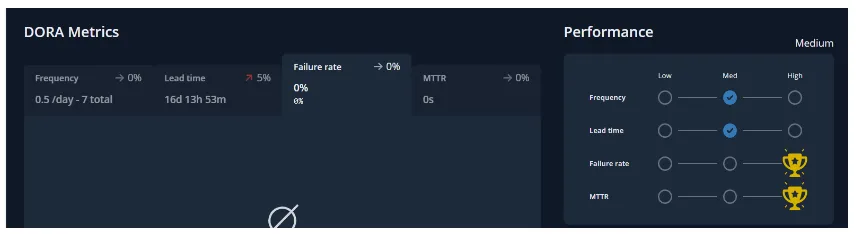
Here’s a table of how DORA metrics are calculated depending on the deployment that occurred, coding and review time, time is taken to restore from an incident or a failure, and failure rate that occurred due to the deployments.
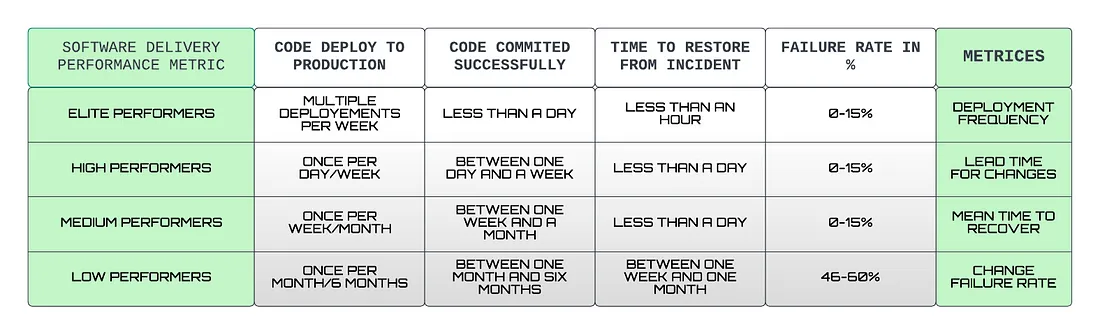
Apart from the four metrics of DORA, there is a fifth one, Reliability, happens to be most important when it comes to operational performance which brings together DevOps and SRE teams to build us a better infrastructure and software. The Reliability metrics is a great way to showcase a team’s overall software delivery performance.
Become an Elite :
According to the most recent State of DevOps report, elite performers have recently grown to now represent 20% of survey respondents. High performers represent 23%, medium performers represent 44%, and low performers only represent 12%.
CONCLUSION :
DORA metrics are a great way to measure the performance of your software development and deployment practices. DORA metrics can help organizations to measure software delivery and stability to a team’s improvement, which also decreases the difficulties and allows for quicker, higher quality software delivery.
Setup of Custom CloudWatch Metrics on your Linux EC2 instance
Amazon CloudWatch can load all the metrics in your account (both AWS resource metrics and application metrics that you provide) for search, graphing, and alarms. Metric data is kept for 15 months, enabling you to view both up-to-the-minute data and historical data.
The CloudWatch monitoring provide some basic monitoring which can be configures in some clicks, while if you want to monitor custom metrics such as the disk and memory utilization of your EC2 machine you should have to follow these steps.
Steps to configure CloudWatch Metrics on Linux Machine:
- Go to AWS Console-> Go To IAM -> Go to Role-> create Role-> Attach CloudWatchAgentServerPolicy -> click next-> Give Role Name-> click create role

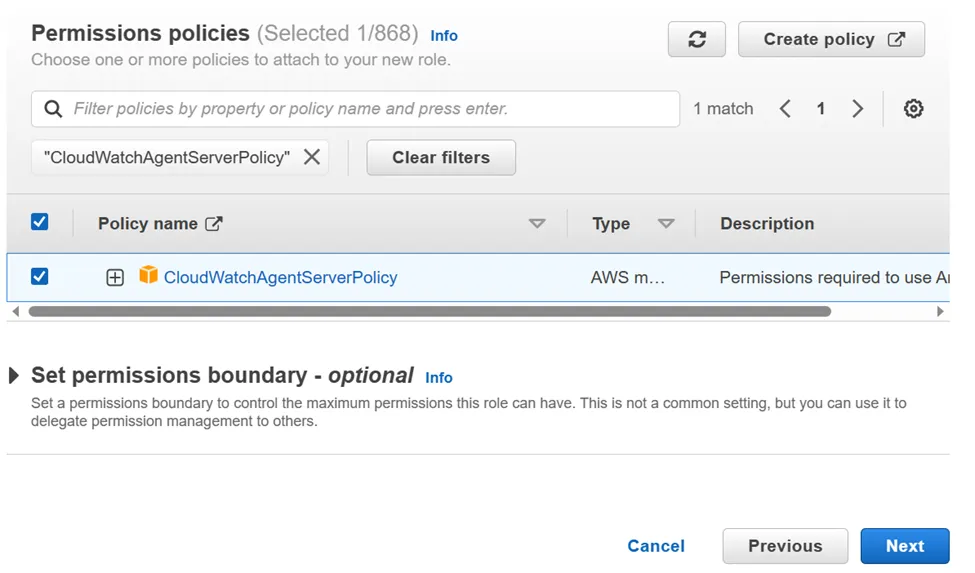
2. Attach created role to the EC2 instance on which you want to do configuration of CloudWatch Metrics.
Go to EC2-> Go to Security-> Go to Modify IAM Role-> Select the Role Name-> click on update IAM Role
3. SSH into Your EC2 instance and apply following Commands
i. wget https://s3.amazonaws.com/amazoncloudwatch-agent/ubuntu/amd64/latest/amazon-cloudwatch-agent.deb
The command will download CloudWatch Agent on your EC2 machine.

iI. sudo dpkg -i -E ./amazon-cloudwatch-agent.deb
The Command will unzip the installed package
iiI. sudo /opt/aws/amazon-cloudwatch-agent/bin/amazon-cloudwatch-agent-config-wizard
The Command start installation of CloudWatch Agent
iv. sudo /opt/aws/amazon-cloudwatch-agent/bin/amazon-cloudwatch-agent-ctl -a fetch-config -m ec2 -c file:/opt/aws/amazon-cloudwatch-agent/bin/config.json -s
The Command will run the AWS CloudWatch Agent



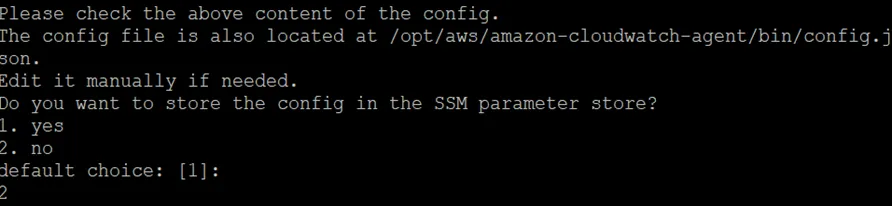
v. sudo /opt/aws/amazon-cloudwatch-agent/bin/amazon-cloudwatch-agent-ctl -a status
The Command Will start the AWS CloudWatch Configuration as per selected Settings.
4. Now monitor the instance from CloudWatch Console.
5. Go to the cloudwatch dashboard->click on all Metrics-> click on cwagent-> click on InstanceId
6. Select Metrics which utilization you want to check

7. This is how you can check the memory, disk and CPU utilization of your EC2 instance.
Kubeflow on AWS

What is Kubeflow?
The Kubeflow project aims to simplify, portability, and scalability of machine learning (ML) workflow deployments on Kubernetes. Our objective is to make it simple to deploy best-of-breed open-source ML systems to a variety of infrastructures, not to replicate other services. Run Kubeflow wherever Kubernetes is installed and configured.
Need of Kubeflow?
The need for Kubeflow arises from the challenges of building, deploying, and managing machine learning workflows at scale. By providing a scalable, portable, reproducible, collaborative, and automated platform, Kubeflow enables organizations to accelerate their machine learning initiatives and improve their business outcomes.
Here are some of the main reasons why Kubeflow is needed:
Scalability: Machine learning workflows can be resource-intensive and require scaling up or down based on the size of the data and complexity of the model. Kubeflow allows you to scale your machine learning workflows based on your needs by leveraging the scalability and flexibility of Kubernetes.
Portability: Machine learning models often need to be deployed across multiple environments, such as development, staging, and production. Kubeflow provides a portable and consistent way to build, deploy, and manage machine learning workflows across different environments.
Reproducibility: Reproducibility is a critical aspect of machine learning, as it allows you to reproduce results and debug issues. Kubeflow provides a way to reproduce machine learning workflows by using containerization and version control.
Collaboration: Machine learning workflows often involve collaboration among multiple teams, including data scientists, developers, and DevOps engineers. Kubeflow provides a collaborative platform where teams can work together to build and deploy machine learning workflows.
Automation: Machine learning workflows involve multiple steps, including data preprocessing, model training, and model deployment. Kubeflow provides a way to automate these steps by defining pipelines that can be executed automatically or manually.
Architecture Diagram:
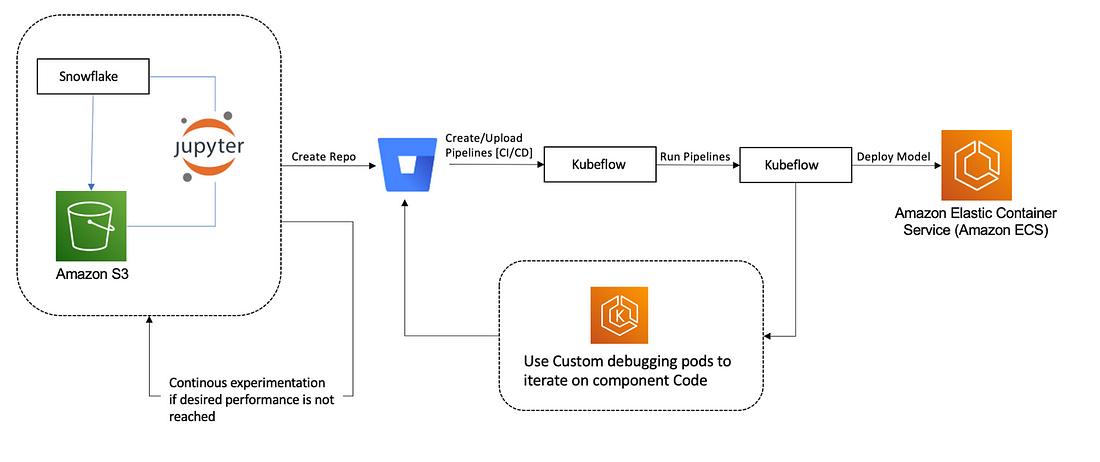
What does Kubeflow do?
Kubeflow provides a range of tools and frameworks to support the entire ML workflow, from data preparation to model training to deployment and monitoring. Here are some of the key components of Kubeflow:
Jupyter Notebooks: Kubeflow includes a Jupyter Notebook server that allows users to run Python code interactively and visualize data in real-time.
TensorFlow: Kubeflow includes TensorFlow, a popular open-source ML library, which can be used to train and deploy ML models.
TensorFlow Extended (TFX): TFX is an end-to-end ML platform for building and deploying production ML pipelines. Kubeflow integrates with TFX to provide a streamlined way to manage ML pipelines.
Katib: Kubeflow includes Katib, a framework for hyperparameter tuning and automated machine learning (AutoML).
Kubeflow Pipelines: Kubeflow Pipelines is a tool for building and deploying ML pipelines. It allows users to define complex workflows that can be run on a Kubernetes cluster.
What is Amazon SageMaker?
Amazon SageMaker is a fully-managed machine learning service that enables data scientists and developers to build, train, and deploy machine learning models at scale. Kubeflow, on the other hand, is an open-source machine learning platform that provides a framework for running machine learning workflows on Kubernetes.
Using Amazon SageMaker with Kubeflow can help streamline the machine learning workflow by providing a unified platform for model development, training, and deployment. Here are the key steps to using Amazon SageMaker with Kubeflow:
Set up a Kubeflow cluster on Amazon EKS or other Kubernetes platforms.
● Install the Amazon SageMaker operator in your Kubeflow cluster. The operator provides a custom resource definition (CRD) that allows you to create and manage SageMaker resources within your Kubeflow environment.
● Use the SageMaker CRD to create SageMaker resources such as training jobs, model endpoints, and batch transform jobs within your Kubeflow cluster.
● Run your machine learning workflow using Kubeflow pipelines, which can orchestrate SageMaker training jobs and other components of the workflow.
● Monitor and manage your machine learning workflow using Kubeflow’s web-based UI or command-line tools.
● By integrating Amazon SageMaker with Kubeflow, you can take advantage of SageMaker’s powerful features for model training and deployment, while also benefiting from Kubeflow’s flexible and scalable machine learning platform.
Amazon SageMaker Components for Kubeflow Pipelines:
Component 1: Hyperparameter tuning job
The first component runs an Amazon SageMaker hyperparameter tuning job to optimize the following hyperparameters:
· learning-rate — [0.0001, 0.1] log scale
· optimizer — [sgd, adam]
· batch-size– [32, 128, 256]
· model-type — [resnet, custom model]
Component 2: Selecting the best hyperparameters
During the hyperparameter search in the previous step, models are only trained for 10 epochs to determine well-performing hyperparameters. In the second step, the best hyperparameters are taken and the epochs are updated to 80 to give the best hyperparameters an opportunity to deliver higher accuracy in the next step.
Component 3: Training job with the best hyperparameters
The third component runs an Amazon SageMaker training job using the best hyperparameters and for higher epochs.
Component 4: Creating a model for deployment
The fourth component creates an Amazon SageMaker model artifact.
Component 5: Deploying the inference endpoint
The final component deploys a model with Amazon SageMaker deployment.
Conclusion:
Kubeflow is an open-source platform that provides a range of tools and frameworks to make it easier to run ML workloads on Kubernetes. With Kubeflow, you can easily build and deploy ML models at scale, while also benefiting from the scalability, flexibility, and reproducibility of Kubernetes.
Please Type Other Keywords
The Ankercloud Team loves to listen
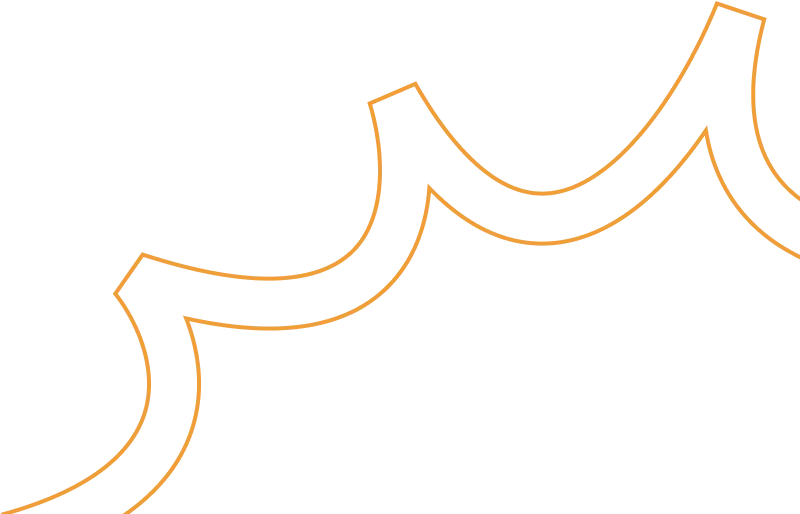
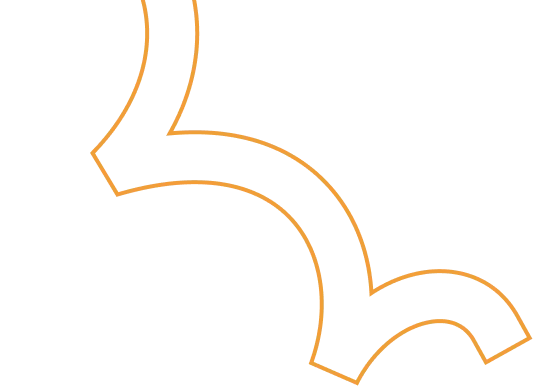